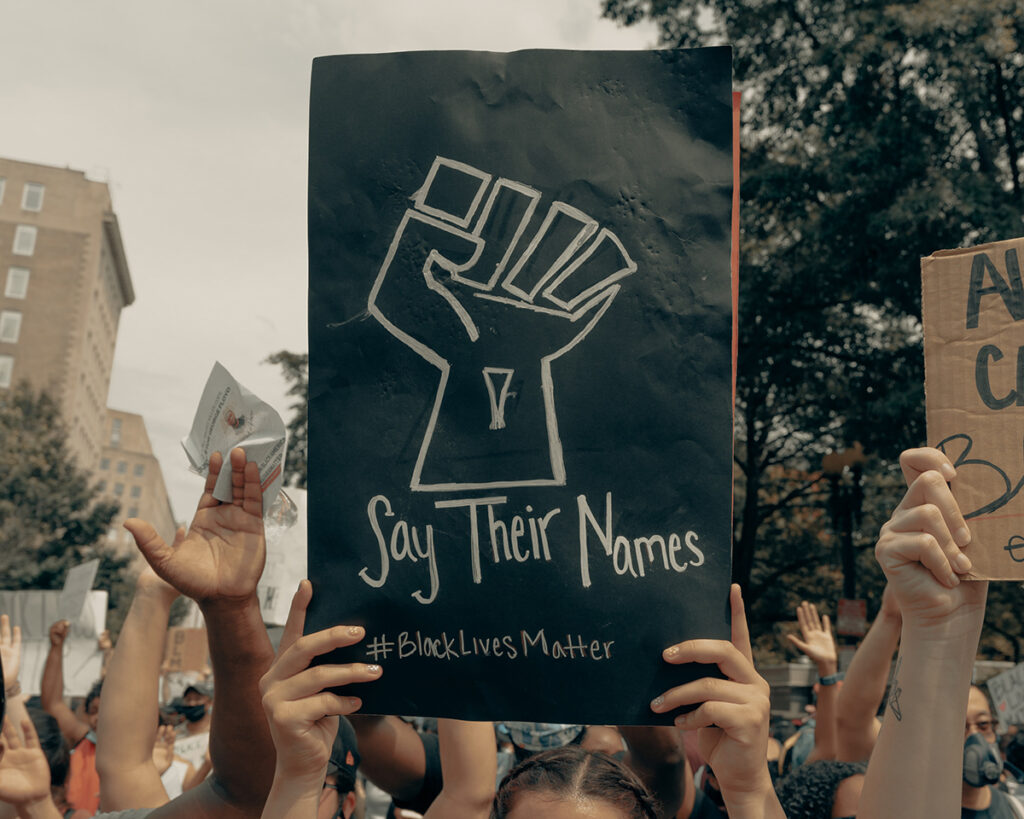
In the spring of 2020, people took to the streets — and to the tweets — in protest after a white police officer murdered George Floyd, a Black man, in Minneapolis by kneeling on his neck and back for over nine minutes. Black Lives Matter, a movement spawned seven years earlier following the shooting death of Trayvon Martin, an unarmed Black teenager, in Florida and the killer’s subsequent acquittal, emerged as the online and offline rallying cry against police brutality and racist violence perpetrated against Black people.
Following Floyd’s death, Twitter users flooded the social media platform with hundreds of millions of posts expressing a range of emotions concerning Black Lives Matter and the campaign for racial justice. A team led by Allen School professor Yulia Tsvetkov sought to facilitate a greater understanding of the connection between those emotional expressions and the narrative surrounding Black Lives Matter and its supporters — and how that connection could also shed light on the role of social media messaging in online activism and large-scale social movements, more generally. To that end, the researchers applied recent advances in natural language processing to analyze the content of 34 million original English-language tweets about BLM posted in 2020 between May 24 and June 28 to identify the prevailing emotions expressed on social media about the movement and associated protests.
The researchers shared their findings in a paper published this week in the Proceedings of the National Academy of Sciences (PNAS) — findings that counter a harmful yet persistent narrative about the emotional tenor of the BLM movement and the people behind it.
“While we identified high levels of anger and disgust across all posts in our dataset, what jumped out at us was the prevalence of positive emotions in posts containing pro-BLM hashtags such as #BlackLivesMatter, #JusticeforFloyd and #NoJusticeNoPeace, and correlating with on-the-ground protests,” said co-lead author and Stanford University postdoc Anjalie Field, who worked on the project as a visiting UW student and Ph.D. candidate at Carnegie Mellon University. “Positive emotions like hope and optimism are more prevalent in posts with explicitly pro-BLM hashtags than other subsets of the data, which contradicts the stereotype of BLM supporters as promoting anger and outrage.”
To perform their analysis, Field, Tsvetkov and their collaborators developed a neural classification model based on the six core emotions identified by psychologist Paul Ekman: anger, disgust, fear, surprise, positivity — what Ekman refers to as “joy” — and sadness. Consistent with prior work applying Ekman’s taxonomy, the researchers treated each category as a superset of finer grained emotions such as the aforementioned hope and optimism (positivity), disapproval and rage (anger), vigilance and apprehension (fear), confusion and curiosity (surprise), and grief and remorse (sadness). Because neural models trained on a pre-collected data set may perform poorly when used to infer meaning from text gathered in a different domain, the team opted for a domain adaptation approach. By combining task-adaptive pre-training with few-shot learning techniques, their methodology permits the re-use of existing annotated datasets to train the model on different domains, rather than going through the effort and expense of collecting and annotating new datasets. They trained their model on two large, pre-existing social media datasets annotated for expressed emotions and adapted it to tweets relating to Black Lives Matter.
As Field noted, anger was the prevailing emotion identified by the model, which detected its presence in 40% or more of the tweets posted over the course of the month. Disgust and positivity alternated as the second-most prevalent emotions, with surprise, sadness and fear rarely approaching 10%. When the researchers analyzed the subset of 6.5 million tweets containing pro-BLM hashtags, they found that positivity consistently outweighed the other categories starting around four days in — on some days, more than double the negative emotions.
Drilling down into the dataset according to date enabled the team to identify instances where emotions spiked, presumably in connection with events. For example, anger and sadness peaked in tweets with pro-BLM hashtags in the days following Floyd’s death and prior to the first weekend of protests. Positivity, meanwhile, rose in the days leading up to that weekend and afterward became the most frequently expressed emotion through the rest of the month. Positivity peaked on the Juneteenth holiday, present in 60% of tweets carrying pro-BLM hashtags. By analyzing location data, the researchers also found that the volume of tweets expressing positive emotions positively correlated with users’ proximity to on-the-ground protests.
Such rich analysis from the team’s adaptive neural model contrasts with that of conventional social science analyses, which typically rely on more rigid lexicon-based approaches using a set list of words associated with an emotion to determine whether content reflects that emotion.
“Lexicon-based models are easy to use, but they aren’t particularly good at capturing broader connotations or adapting to new contexts,” explained co-lead author Chan Young Park, a visiting UW student who is pursuing a Ph.D. from CMU. “For example, one popular model connotes the word ‘police’ with the terms ‘fear,’ ‘positive’ and ‘trust’ — emotions that are unlikely to factor into protests against police brutality. Our framework offers a more robust method for extracting accurate social meaning from text data that can be adapted to different contexts and language varieties.”
The meaning the team extracted from posts using its computational model is consistent with findings from previous social psychology studies; while moral outrage and anger can prompt people to become involved in social movements, positive emotions are necessary to sustain that involvement over time.
“Words and emotions are powerful tools for online activism. Emerging NLP techniques are also powerful tools that can help us understand how those words and emotions contribute to building and sustaining social movements,” said Tsvetkov. “In doing so, they can help us also to dispel negative stereotypes about marginalized communities that lead to physical, social and economic harm.”
Additional contributors to the paper include co-lead author and then-student Antonio Theophilo, who recently earned his Ph.D. from the Institute of Computing at the University of Campinas in Brazil, and Jamelle Watson-Daniels, a Ph.D. student at Harvard University and Director of Research at Data for Black Lives.