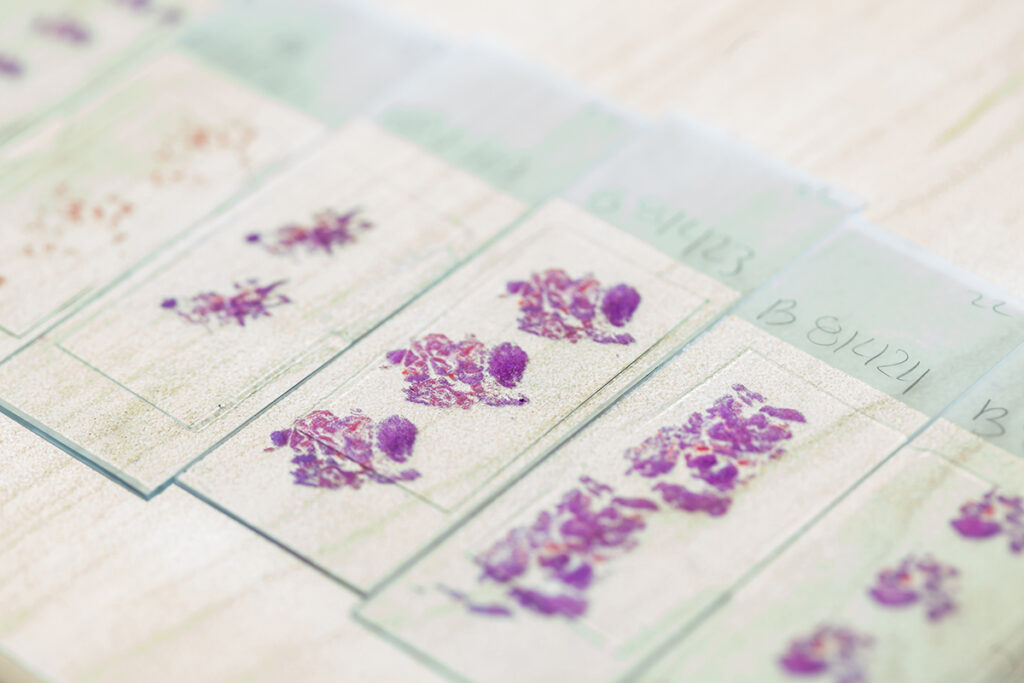
Digital pathology promises to revolutionize medicine by transforming tissue samples into high-resolution images that can be shared and analyzed using powerful new computational tools. Instead of looking at single slides through a microscope, scientists and clinicians can analyze vast quantities of tissue samples at once, identifying anomalies, searching for patterns, and — thanks to advances in artificial intelligence — making predictions about various disease characteristics to assist with clinical decision-making and personalize patient care.
But there are multiple challenges that have to be overcome to achieve this bold new vision for medicine, from the scarcity of real-world data to the amount of compute required for model pretraining. Now, thanks to researchers at the University of Washington’s Allen School, Microsoft Research and Providence, the prospects for digital pathology are looking even brighter — around a billion times brighter. In a paper recently published in the journal Nature, the team unveiled Prov-GigaPath, a groundbreaking new open-access foundation model for digital pathology that combines real-world, whole-slide data with individual image tiles at an unprecedented scale.
Prov-GigaPath was developed with what the team believes to be the largest whole-slide pretraining effort to date, using samples collected from over 30,000 cancer center patients in the Providence health network. Prov-Path — a portmanteau of “Providence” and “pathology” — includes more than 1.3 billion image tiles derived from roughly 170,000 whole pathology slides. That’s five times larger than another whole-slide dataset, The Cancer Genome Atlas (TCGA), and is drawn from twice the number of patients. And whereas TCGA contains only tissue resections, the data underpinning Prov-GigaPath includes both resections and biopsies covering 31 major tissue types.
According to co-first author and Allen School Ph.D. student Hanwen Xu, the scale of the inputs, coupled with finer details about the samples, gives Prov-GigaPath the edge when it comes to potential integration with clinical workflows.
“The Prov-Path dataset is both really diverse and really robust, including meta information such as genomic mutation profiles, longitudinal data and real-world pathology reports,” explained Xu, “Those details provide us with a great opportunity to develop stronger models that can handle a wide range of real-world tasks, like tumor detection, cancer staging, and mutation prediction.”
The researchers developed Prov-GigaPath using an efficient vision transformer architecture, GigaPath, that was built on a version of LongNet’s dilated attention mechanism. This approach allowed for efficient aggregation of the tens of thousands of image tiles contained in a single slide, thus significantly lowering the computational cost for pretraining compared to the standard transformer model. It also enables Prov-GigaPath to pick out patterns other models cannot by embedding image tiles as visual tokens; not only is it trained on the characteristics of the individual tokens, but it can also detect patterns across a sequence of tokens that corresponds to an entire slide.
“The key technical challenge is that whole-slide pathology images are extremely large compared to other images studied in the computer vision domain. A pathology image could be as large as 120,000 by 120,000 pixels,” noted Allen School professor and co-corresponding author Sheng Wang. “On one hand, it is challenging for a pathologist to review the entire slide, necessitating the development of automated AI approaches. On the other hand, it is challenging for existing generative AI models, which are often transformer-based, to be scaled to sequences from such large images due to the computational burden.
“We address this problem by using a new neural network architecture that can scale to very long sequences,” Wang continued. “As a result, Prov-GigaPath makes predictions based on global patterns as well as localized ones, which yields state-of-the-art performance on multiple prediction tasks.”
Those tasks range from standard histopathology tasks like cancer subtyping to more challenging tasks like mutation prediction. In all, Wang and his colleagues evaluated Prov-GigaPath on a set of 26 prediction tasks, comparing their new model’s performance against that of other digital pathology foundation models such as HIPT and REMEDIS trained on more limited datasets. Prov-GigaPath outperformed them all on 25 out of the 26 tasks — even when the competing model was pretrained on the same dataset used for the task and Prov-GigaPath was not. The new model also set a new benchmark for predicting cancer subtypes from images, outperforming other models on each of nine cancer types; for six of the subtypes, the performance improvement over the next-best model was significant.
According to Xu, these results point to the potential utility of the team’s approach for a variety of downstream tasks — for cancer, and beyond.
“With Prov-GigaPath, we showed how to build a model that can do representation learning of high-resolution image data efficiently at a really large scale,” said Xu. “I’m excited to see how researchers will take this base model and apply it to other biomedical problems to advance AI-assisted diagnostics and decision support.”
Researchers and clinicians can freely access Prov-GigaPath on Hugging Face. Going forward, Xu and Wang hope to extend this approach to other imaging data, such as that derived very large microscopy images for proteins and single cells. They are actively seeking pathologists and physicians within UW Medicine who would like to collaborate on this effort, particularly those who are interested in using the existing model to analyze their data or those with large amounts of imaging data that the team could leverage to train a new model.
In addition to Wang and Xu, contributors to Prov-GigaPath include co-first author Naoto Usuyama, co-corresponding author Hoifung Poon, and their Microsoft colleagues Jaspreet Bagga, Sheng Zhang, Rajesh Rao, Tristan Naumann, Cliff Wong, Zelalem Gero, Javier González Hernández, Yu Gu, Yanbo Xu, Mu Wei, Wenhui Wang, Shuming Ma, Furu Wei, Jianwei Yang, Chunyuan Li and Jianfeng Gao; co-corresponding author Carlo Bifulco and Brian Piening of Providence Cancer Institute and Providence Genomics; Jaylen Rosemon and Tucker Bower of Providence Genomics; and Soohee Lee, Roshanthi Weerasinghe, Bill J. Wright and Ari Robicsek of the Providence Research Network.
To learn more, see the Nature paper, Microsoft Research blog post, and Providence announcement and Q&A. Also check out related coverage by GeekWire, FierceBiotech and Forbes.