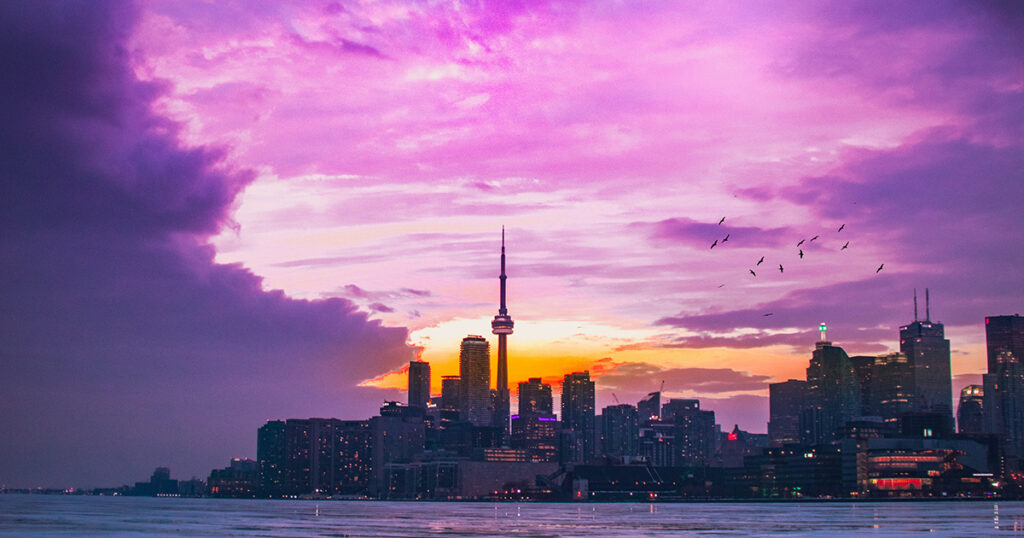
Allen School researchers took home multiple Best Paper and Outstanding Paper Awards from the 61st Annual Meeting of the Association for Computational Linguistics (ACL) held in Toronto last month. Their research spanned a number of projects aimed at enhancing the performance and impact of natural language models, including how artificial intelligence (AI) processes humor, the impact of built-in political biases on model performance, AI-assisted cognitive reframing to support mental health, identifying “WEIRD” design biases in datasets and how to imbue language models with theory of mind capabilities. Read more about their contributions below.
Best Paper Awards
Do Androids Laugh at Electric Sheep? Humor ‘Understanding’ Benchmarks from The New Yorker Caption Contest
Allen School professor Yejin Choi and her collaborators earned a Best Paper Award for their study exploring how well AI models understand humor, challenging these models with three tasks involving The New Yorker Cartoon Caption Contest.
The tasks included matching jokes to cartoons, identifying a winning caption and explaining why an image-caption combination was funny. For an AI model, it’s no joke. Humor, the authors point out, contains “playful allusions” to human experience and culture. Its inherent subjectivity makes it difficult to generalize, let alone explain altogether.
“Our study revealed a gap still exists between AI and humans in ‘understanding’ humor,” said Choi, who holds the Wissner-Slivka Chair at the Allen School and is also senior research manager for the Allen Institute for AI’s MOSAIC project. “In each task, the models’ explanations lagged behind those written by people.”
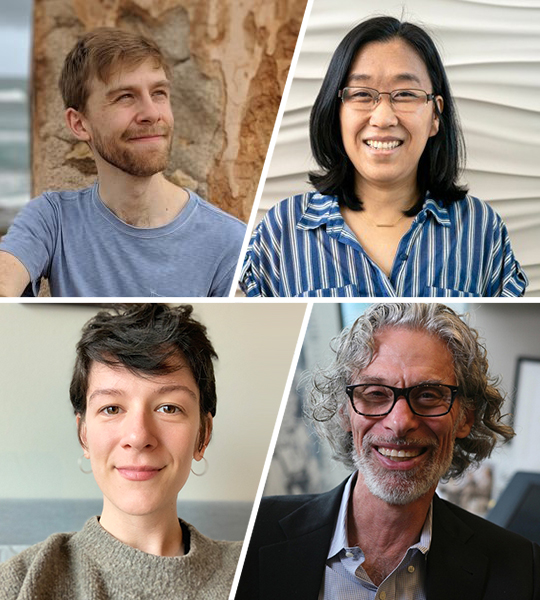
The team applied both multimodal and language-only models to the caption data. Compared to human performance, the best multimodal models scored 30 accuracy points worse on the matching task.
Even the strongest explanation model, GPT-4, fell behind. In more than two-thirds of cases, human-authored explanations were preferred head-to-head over the best machine-authored counterparts.
Future studies could focus on other publications or sources. The New Yorker Cartoon Caption Contest represents only a “narrow slice” of humor, the authors note, one that caters to a specific audience. New research could also explore generating humorous captions by operationalizing feedback produced by the team’s matching and ranking models.
The study’s authors also included Jack Hessel and Jena D. Hwang of AI2, professor Ana Marasović of the University of Utah, professor Lillian Lee of Cornell University, Allen School alumni Jeff Da (B.S., ‘20) of Amazon and Rowan Zellers (Ph.D., ‘22) of OpenAI and Robert Mankoff of Air Mail and Cartoon Collections.
From Pretraining Data to Language Models to Downstream Tasks: Tracking the Trails of Political Biases Leading to Unfair NLP Models
Allen School professor Yulia Tsvetkov, Ph.D. student Shangbin Feng and their collaborators earned a Best Paper Award for their work focused on evaluating pretrained natural language processing (NLP) models for their political leanings and using their findings to help combat biases in these tools.
To do this, they developed a framework based on political science literature for measuring bias found in pretrained models. Then they analyzed how these biases affected the models’ performance in downstream social-oriented tasks, such as measuring their ability to recognize hate speech and misinformation.
They found that bias and language are difficult to separate. Both non-toxic and non-malicious data, they note, can cause biases and unfairness in NLP tasks. If political opinions are filtered from training data, however, then questions arise concerning censorship and exclusion from political participation. Neither is an ideal scenario.
“Ultimately, this means that no language model can be entirely free from social biases,” Tsvetkov said. “Our study underscores the need to find new technical and policy approaches to deal with model unfairness.”
The consistency of the results surprised the team. Using data from Reddit and several news sources, the researchers found that left-leaning and right-leaning models acted according to form. The left-leaning models were better at detecting hate speech towards minority groups, while worse at detecting hate speech towards majority groups. The pattern was reversed for right-leaning models.
In evaluating data from two time periods — before and after the 2016 U.S. presidential election — they also discovered a stark difference between the levels of political polarization and its attendant effect on the models’ behavior. With more polarization comes more bias in language models.
Future studies could focus on getting an even more fine-grained picture of the effect of political bias on NLP models. For example, the authors note that being liberal on one issue does not preclude being conservative on another.
“There’s no fairness without awareness,” Tsvetkov said. “In order to develop ethical and equitable technologies, we need to take into account the full complexity of language, including understanding people’s intents and presuppositions.”
The study’s co-authors also included Chan Young Park, a visiting Ph.D. student from Carnegie Mellon University, and Yuhan Liu, an undergraduate at Xi’an Jiaotong University.
Outstanding Paper Awards
Cognitive Reframing of Negative Thoughts through Human-Language Model Interaction
Ph.D. students Ashish Sharma and Inna Wanyin Lin and professor Tim Althoff, director of the Allen School’s Behavioral Data Science Group, were part of a team that won an Outstanding Paper Award for their project investigating how language models can help people reframe negative thoughts and what linguistic attributes make this process effective and accessible.
Working with experts at Mental Health America, the team developed a model that generates reframed thoughts to support the user. For example, the model could produce reframes that were specific, empathic or actionable — all ingredients for a “high-quality reframe.” The study was the first to demonstrate that these all make for better reframes, Althoff said, and the team illustrated this with gold standard randomized experiments and at scale.
The research has already seen real-world impact. Since its introduction late last year, the team’s reframing tool has had more than 60,000 users.
“The findings from this study were able to inform psychological theory — what makes a reframe particularly effective?” Sharma said. “Engaging with real users helped us assess what types of reframes people prefer and what types of reframes are considered relatable, helpful and memorable.”
Those “high-quality reframes” could be particularly helpful for those who lack access to traditional therapy. The team pointed out several obstacles to care, including clinician shortages, lack of insurance coverage and stigmas surrounding mental health, that served as motivations for the study.
The model can also be integrated into existing therapy workflows, Sharma added, helping both clients and therapists in the process. For example, therapists often assign “homework” to clients, asking them to practice cognitive reframing, a technique by which a person can picture a negative thought through a different, more balanced perspective.
But many clients report having difficulty in applying those techniques following a session. Sharma and Althoff said the team’s reframing tool can provide support in those moments.
“It turns out that often our thoughts are so deep-rooted, automatic and emotionally triggering that it can be difficult to reframe thoughts on our own,” Althoff said. “This kind of research not only helps us improve our intervention itself, but could also inform how clinicians teach these skills to their clients.”
The study’s co-authors also included Kevin Rushton, Khendra G. Lucas and Theresa Nguyen of Mental Health America, Allen School alum David Wadden (Ph.D., ‘23) of AI2 and Stanford University professor and clinical psychologist Adam S. Miner.
Minding Language Models’ (Lack of) Theory of Mind: A Plug-and-Play Multi-Character Belief Tracker
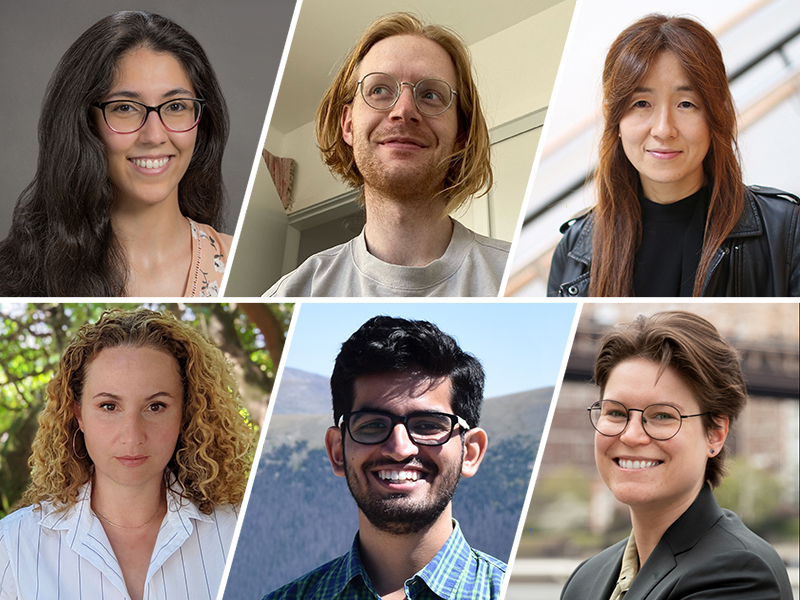
Choi and Tsvetkov worked with Allen School Ph.D. students Melanie Sclar and Peter West and collaborators on SymbolicToM, an algorithm that improves large language models’ abilities to reason about the mental states of other people that earned the team an Outstanding Paper Award. The team also won the Outstanding Paper Award at the ToM Workshop at the 2023 International Conference on Machine Learning (ICML) for this work.
Theory of mind (ToM), or the ability to reason about others’ thoughts and intentions, is a key part of human intelligence. But today’s AI models lack ToM capabilities out of the box. Prior efforts at integrating ToM into language models required training, with existing reading comprehension datasets used for ToM reasoning remaining too simplistic and lacking diversity.
“This implied that models trained solely on this data would not perform ToM reasoning,” Sclar said, “and rather only mimic these skills for the simplistic data they were trained on.”
Enter SymbolicToM. Without requiring ToM-specific training, the decoding-time algorithm takes a divide-and-conquer approach. SymbolicToM splits a given problem into subtasks, Sclar said, solving each with off-the-shelf large language models. The result is a better, more robust model.
“We knew from the get-go that our approach needed to focus on having good generalization capabilities, and thus would benefit from not requiring training,” Sclar said. “SymbolicToM is to the best of our knowledge the first method for theory of mind reasoning in natural language processing that does not require any specific training whatsoever.”
The team tasked SymbolicToM with answering reading comprehension questions based on a story featuring multiple characters. They tracked each character’s beliefs, their estimation of others’ beliefs and higher-order levels of reasoning through graphical representations. In doing so, the models could reason with more precision and interpretability.
“Our method in particular is not focused on training neural language models, but quite the opposite: given that we have imperfect language models trained with other objectives in mind, how can we leverage them to dramatically improve theory of mind performance?” Tsvetkov said. “This is key because data with explicit theory of mind interactions are scarce, and thus training directly is not a viable option.”
Sclar pointed to potential avenues for future applications, including education and business. For example, AI agents with ToM reasoning skills could assist in tutoring applications, providing a deeper understanding of students’ knowledge gaps and designing tests based on their mental model of each student.
Another instance involves negotiation strategy. If AI agents can intuit what each party hopes to achieve and how much they value certain aspects of a deal, Sclar said, they can provide support in reaching a fair consensus.
“Imbuing neural language models with ToM capabilities would improve these models’ potential on a wide range of applications,” Sclar said, “as well their understanding of human interactions.”
The study’s authors also included visiting Ph.D. student Sachin Kumar of Carnegie Mellon University and professor Alane Suhr of the University of California, Berkeley.
NLPositionality: Characterizing Design Biases of Datasets and Models
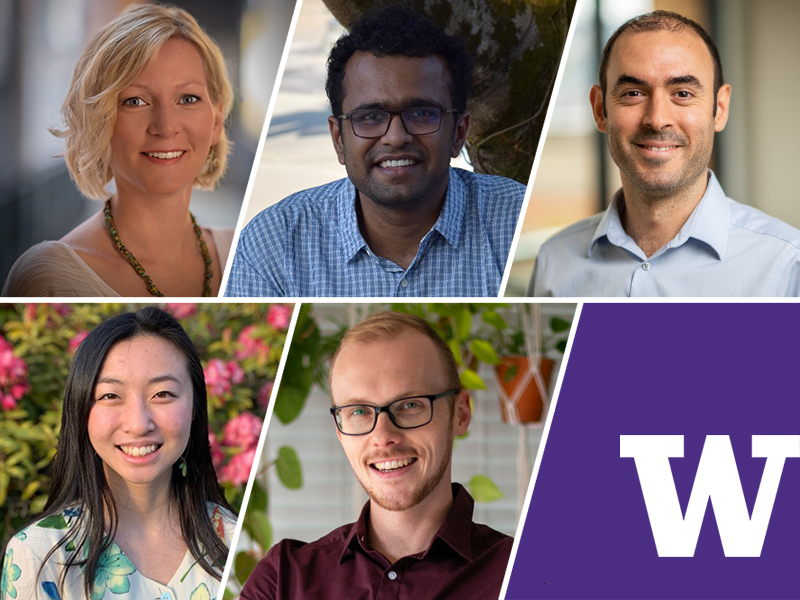
Ph.D. student Sebastin Santy, professor Katharina Reinecke and their collaborators won an Outstanding Paper Award for devising a new framework for measuring design biases and positionality in NLP datasets that provides a deeper understanding of the nuances of language, stories and the people telling them.
“Language is a social phenomenon,” Santy said. “Many in the NLP field have noticed how certain datasets and models don’t work for different populations, so we felt it was the right time to conduct this large-scale study given these gaps and with the right kind of platform.”
That platform, LabintheWild, provides more reliable data from a more diverse set of users. Reinecke, one of the platform’s co-founders and director of the Wildlab at the Allen School, noted that as opposed to Mechanical Turk, a popular paid crowdsourcing site, LabintheWild collects results from a greater pool of countries.
With LabintheWild, the personal is emphasized over the pecuniary. After completing a study, users can see personalized feedback and compare their results with others’ performance on the platform.
This feedback is eminently shareable, Reinecke added, increasing its reach. The researchers’ recent study collected 16,299 annotations from 87 countries — one of the first NLP studies to reach that scale. They applied their framework, called NLPositionality, to LabintheWild’s vast participant pool, implementing users’ annotations from existing datasets and models for two tasks: social acceptability and hate speech detection.
Their findings aligned with Reinecke’s previous work, which shows that technology is often designed for people who are Western, Educated, Industrialized, Rich and Democratic, or “WEIRD.”
“WEIRD bias is well-known in psychology and our hypothesis was that we might find similar results in AI as well, given most of the recent advances make use of mostly English data from the internet and filter for ‘high-quality,’ ” said Reinecke, who holds the Paul G. Allen Career Development Professorship. “While we had a feeling that there would be Western bias because of how most of the datasets are curated in the Western Hemisphere, we did not expect it to be this pronounced.”
The study’s co-authors also included Allen School alumni Maarten Sap (Ph.D., ‘21) and Jenny Liang (B.S., ‘21), now professor and Ph.D. student, respectively, at Carnegie Mellon University, and Ronan Le Bras of AI2.