While the Allen School’s annual Research Showcase and Open House highlights both the breadth and depth of computing innovation at the state’s flagship university, the 2024 event at the University of Washington last week had a decidedly AI flavor. From a presentation on advances in AI for medicine, to technical sessions devoted to topics such as safety and sustainability, to the over 100 student research projects featured at the evening poster session, the school’s work to advance the foundations of AI and its ever-expanding range of applications took center stage.
“Medicine is inherently multimodal”
In his luncheon keynote on generative AI for multimodal biomedicine, Allen School professor Sheng Wang shared his recent work towards building foundation models that bring together medical imaging data from multiple sources — such as pathology, X-ray and ultrasound — to assist doctors with diagnosing and treating disease.
“Medicine is inherently multimodal,” noted Wang. “There are lots of complicated diseases, like diabetes, hypertension, cancer, Alzheimer’s or even Covid…and we will see signals all over the body.”
The ability to capture these signals using multiple imaging modalities requires overcoming a number of challenges. For example, pathology images are too large for existing AI models to analyze in sufficiently detailed resolution — 100,000 by 100,000 pixels, large enough to cover a tennis court. Typically, images encountered by AI models are closer to 256 by 256 pixels which, in keeping with Wang’s analogy, is akin to a single tennis ball.
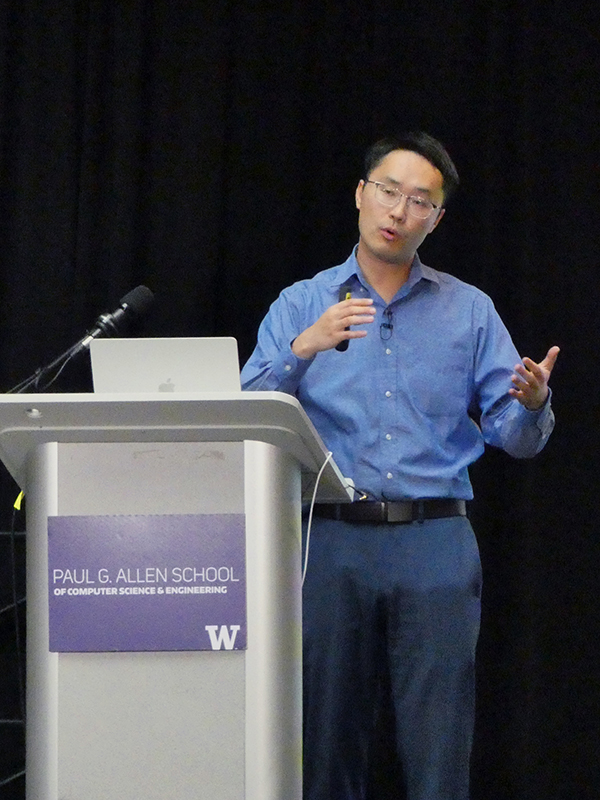
To make pathology images more manageable, Wang and his collaborators looked to generative AI. Despite the stark difference in domains, “the challenge or the solution here is very similar to the underlying problem behind ChatGPT,” Wang explained. ChatGPT can understand and summarize long documents; by converting large pathology slide images to a “long sentence” of smaller images, Wang and his colleagues determined AI could then summarize these image-sentences to obtain an overview of a patient’s status. Based on that idea, Wang and his team developed GigaPath, the first foundation model for whole-slide pathology. GigaPath, which achieved state-of-the-art performance on 25 out of 26 tasks, is “one model fits all,” meaning it can be applied to different types of cancer. Since its release, the tool is averaging 200,000 downloads per month.
One task for which AI models typically do not perform well is predicting which treatment to recommend for a particular patient. So Wang and his colleagues borrowed another concept drawn from generative AI, chain-of-thought, which calls for decomposing a complicated task into multiple, small subtasks. The model is then asked to solve those smaller tasks individually on the way to addressing the bigger, more challenging task.
“The question is, how can we apply chain-of-thought to medicine?” Wang asked. “This has never been done before.” The answer is to use clinical guidelines as the chain to instruct a large language model (LLM). By breaking the chain into subtasks such as predicting cancer subtype and patient biomarkers, the LLM then arrives at a prediction of the appropriate treatment.
Yet another challenge is how to apply AI to 3D medical imaging. Here again, Wang and his colleagues achieved a milestone by developing the first 3D OCT foundation model. OCT is short for optical coherence tomography, a type of imaging used to diagnose retinal diseases.
“Our model can comprehensively understand the entire 3D structure to make a diagnosis,” said Wang, who aims to extend this approach to other types of medical 3D imaging, like MRI and CT scans — and eventually, to create one model that can handle everything. This is challenging for even general domain machine learning; the state of the art, CLIP, is limited to two modalities, Wang noted; he wants to build a medical model that can integrate as many as nine.
To overcome the problem, Wang and his fellow researchers drew inspiration from Esperanto, a constructed language that provides a common means of communication among a group of people who speak different languages. They devised an approach, BiomedParse, in which they built one foundation model for each modality, and then projected everything into the medical imaging equivalent of Esperanto — in this case, human language in the form of text from the associated clinical reports — as the common space into which they can project the millions of images, both 2D and 3D, from the different modalities.
But Wang wants to go beyond multi-modal to multi-agent. Using the example of a molecular tumor board, in which multiple experts convene to discuss challenging cases to determine a course of treatment, he suggested that AI models developed for different imaging modalities could help doctors efficiently and accurately determine a treatment plan — analogous to a Microsoft 365 for cancer research. And while some doctors may worry about AI replacing them, Wang’s approach is focused on advancing human-AI collaboration: Medical experts still develop the high-level guidelines for the model, with the AI handling the individual steps.
“In the future the AI model could be like a clinical lab test every doctor can order,” Wang suggested. “The doctor can order an AI test to do a specific task, and then the doctor will make a decision based on the AI output.”
“It’s just really exciting to see all this great work”
The event culminated with the announcement of the recipients of the Madrona Prize, which is selected by local venture capital firm and longtime Allen School supporter Madrona Venture Group to recognize innovative research at the Allen School with commercial potential. Rounding out the evening was the presentation of the People’s Choice Award, which is given to the team with the favorite poster or demo as voted on by attendees during the event — or in this case, their top two.
Managing Director Tim Porter presented the Madrona Prize, which went to one winner and two runners up. Noting that previous honorees have gone on to raise hundreds of millions of dollars and get acquired by the likes of Google and Nvidia, he said, “It’s just really exciting to see all this great work turning into things that have long-term impact on the world through commercial businesses and beyond.”
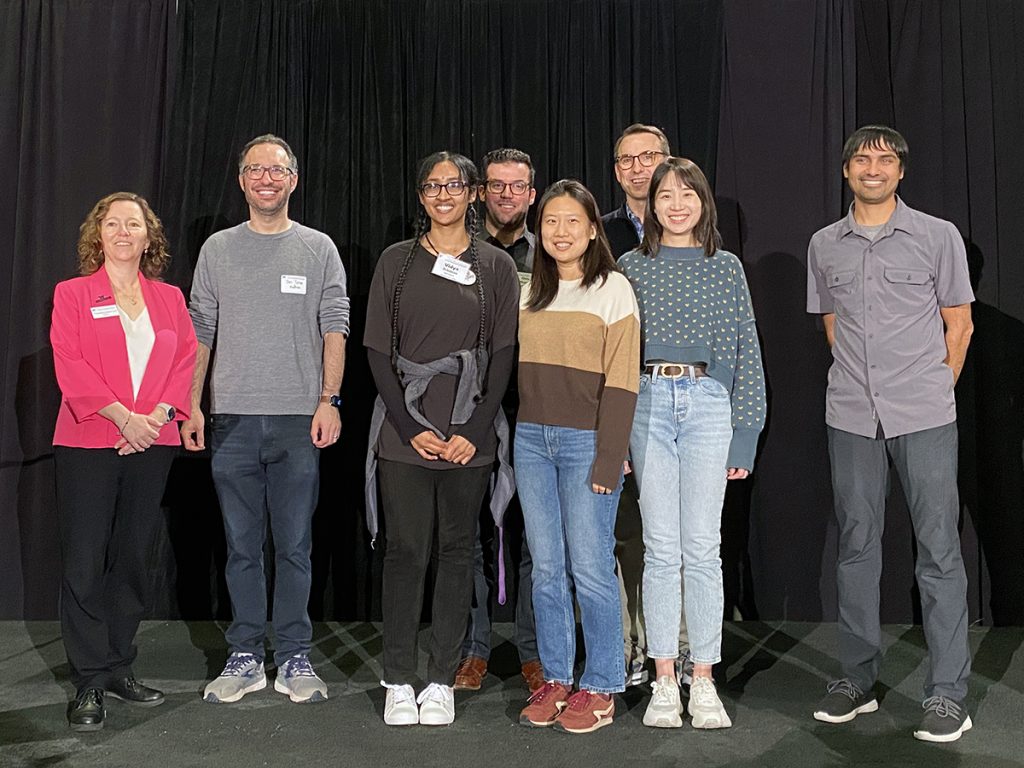
Madrona Prize winner / Designing AI systems to support team communication in remote work
Allen School Ph.D. student Ruotong Wang accepted Madrona’s top prize for a pair of projects that aim to transform workplace communication — Meeting Bridges and PaperPing.
The Covid-19 pandemic has led to a rise in remote meetings, as well as complaints of “Zoom fatigue” and “collaboration overload.” To help alleviate this negative impact on worker productivity, Wang proposed meeting bridges, or information artifacts that support post-meeting collaboration and help shift work to periods before and after meetings. Based on surveys and interviews with study participants, the team devised a set of design principles for creating effective meeting bridges, such as the incorporation of multiple data types and media formats and the ability to put information into a broader context.
Meanwhile, PaperPing supports researcher productivity in the context of group chats by suggesting papers relevant to their discussion based on social signals from past exchanges, including previous paper citations, comments and emojis. The system is an implementation of Social-RAG, an AI agent workflow based on the concept of retrieval-augmented generation that feeds the context of prior interactions among the group’s members and with the agent itself into a large language model (LLM) to explain its current recommendations.
Additional authors on Meeting Bridges include Allen School alum Lin Qui (B.S. ‘23) and professor Amy Zhang, as well as Maestro AI co-founder Justin Cranshaw. In addition to Zhang and Qui, Allen School postdoc Xinyi Zhou and Allen Institute for AI’s Joseph Chee Chang and Jonathan Bragg (Ph.D., ‘18) contributed to PaperPing.
Madrona Prize runner up / Interpreting nanopore signals to enable single-molecule protein sequencing
For one of two runners up, Madrona singled out a team of researchers in the Allen School’s Molecular Information Systems Laboratory (MISL) for developing a method for long-range, single-molecule protein sequencing using commercially available nanopore sensing devices from Oxford Nanopore Technologies. Determining protein sequences, or the order that amino acids are arranged within a protein molecule, is key to understanding their role in different biological processes. This technology could help researchers develop medications targeting specific proteins for the treatment of cancer and neurological diseases such as Alzheimer’s.
The research team includes Allen School Ph.D. students Daphne Kontogiorgos-Heintz and Melissa Queen, current Master’s student Sangbeom Yang (B.S., ‘24), former postdoc Keisuke Motone, now a faculty member at Osaka University, and research professor Jeff Nivala; MISL undergraduate researchers Jasmine Wee, Yishu Fang and Kyoko Kurihara, lab manager Gwendolin Roote and research scientist Oren E. Fox; UW Molecular Engineering and Science Institute Ph.D. student Mattias Tolhurst and alum Nicolas Cardozo; and Miten Jain, now a professor of bioengineering and physics at Northeastern University.
Madrona Prize runner up / Knowledge boosting during low-latency inference
Another team of researchers earned accolades for their work on knowledge boosting, a technique for bridging potential communication delays between small AI models running locally on edge devices and larger, remote models to support low-latency applications. This approach can be used to improve the performance of a small model operating on headphones, for example, with the help of a larger model running on a smartphone or in the cloud. Potential uses for the technology include noise cancellation features, augmented reality and virtual reality headsets, and other mobile devices that run AI software locally.
Lead author Vidya Srinivas accepted the award on behalf of the team, which includes fellow Allen School Ph.D. student Tuochao Chen and professor Shyam Gollakota; Malek Itani, a Ph.D. student in the UW Department of Electrical & Computer Engineering; Microsoft Principal Researcher Emre Sefik Eskimez and Director of Research at AssemblyAI Takuya Yoshioka.
People’s Choice Award (tie) / AHA: A vision-language-model for detecting and reasoning over failures in robotic manipulation
Attendees could not decide on a single favorite presentation of the night, leading to a tie for the People’s Choice Award.
While advances in LLMs and vision-language models may have expanded robots’ problem solving, object recognition and spatial reasoning capabilities, they’re lacking when it comes to recognizing and reasoning about failures — which hinders their deployment in dynamic, real-world settings. The research team behind People’s Choice honoree AHA: A Vision-Language-Model for Detecting and Reasoning over Failures in Robotic Manipulation designed an open-source VLM that identifies failures and provides detailed natural-language explanations for those failures.
“Our work focuses on the reasoning aspect of robotics, often overlooked but essentially especially with the rise of multimodal large language models for robotics,” explained lead author and Allen School Ph.D. student Jiafei Duan. “We explore how robotics could benefit from these models, particularly by providing these models with the capabilities to reason about failures in the robotics execution and hence helped with improving the downstream robotic systems.”
Using a scalable simulation framework for demonstrating failures, the team developed AHA to effectively generalize to a variety of robotic systems, tasks and environments. Duan’s co-authors include Allen School Ph.D. student Yi Ru Wang, alum Wentao Yuan (Ph.D. ‘24) and professors Ranjay Krishna and Dieter Fox; Wilbert Pumacay, a Master’s student at the Universidad Católica San Pablo; Nishanth Kumar, Ph.D. student at the Massachusetts Institute of Technology; Shulin Tian, an undergraduate researcher at Nanyang Technological University; and research scientists Ajay Mandlekar and Yijie Guo of Nvidia.
People’s Choice Award (tie) / AltGeoViz: Facilitating accessible geovisualization
The other People’s Choice Award winner was AltGeoViz, a system that enables screen-reader users to explore geovisualizations by automatically generating alt-text descriptions based on the user’s current map view. While conventional alt-text is static, AltGeoViz dynamically communicates visual information such as viewport boundaries, zoom levels, spatial patterns and other statistics to the user in real time as they navigate the map — inviting them to interact with and learn from the data in ways they previously could not.
“Coming from an urban planning background, my motivation for pursuing a Ph.D. in human-computer interaction originates from my passion for helping people design better cities,” lead author and Allen School Ph.D. student Chu Li said. “AltGeoViz represents a step towards this goal — by making spatial data visualization accessible to blind and low-vision users, we can enable broader participation in the urban planning process and shape more inclusive environments.”
Li’s co-authors include Allen School Ph.D. students Rock Yuren Pang, Ather Sharif and Arnavi Chheda-Kothary and professors Jeffrey Heer and Jon Froehlich.
For more about the Allen School’s 2024 Research Showcase and Open House, read GeekWire’s coverage of the daytime sessions here and the award winners here, and Madrona Venture Group’s announcement here.
Kristine White contributed to this story.