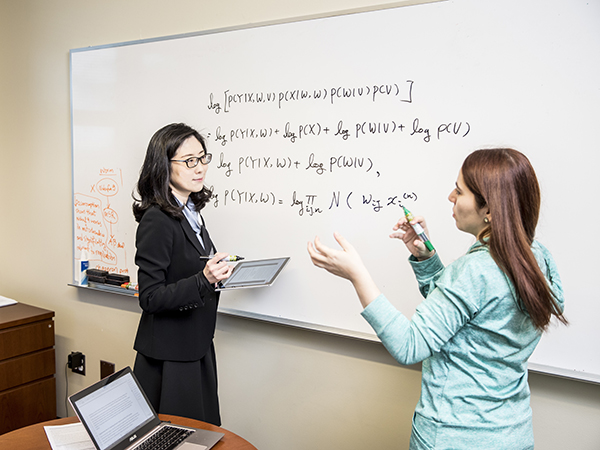
Allen School and Genome Sciences professor Su-In Lee, left, and Allen School Ph.D. student Safiye Celik discuss the key formula for their MERGE algorithm to match patients with cancer drugs based on their molecular profiles. Credit: Dennis Wise
In the latest example of computing’s potential to transform health care, a team of researchers at the Allen School, UW Department of Genome Sciences, and UW Medicine is applying a combination of machine learning and big data to improve outcomes for cancer patients. The team’s approach, detailed in a new paper published in the journal Nature Communications, helps physicians deliver targeted treatment to patients based on their individual molecular profiles.
Allen School and Genome Sciences professor Su-In Lee, first and corresponding author, and Allen School Ph.D. candidate Safiye Celik, co-first author, have developed a new machine learning algorithm called MERGE to identify reliable biomarkers of therapeutic response to 160 anti-cancer drugs in cases of acute myeloid leukemia (AML) — an aggressive form of cancer of the blood and bone marrow cells that is predominantly found in older adults — in collaboration with UW Hematology and Institute for Stem Cell & Regenerative Medicine professors Pamela S. Becker and C. Anthony Blau. MERGE “recycles” publicly available genomic data from many AML patients and biological knowledge databases to find the best drugs for AML patients and opens promising avenues to improve patient care for many other diseases in the era of precision medicine.
MERGE — short for “Mutation, Expression hubs, known Regulators, Genomic CNV, and mEthylation” — is a novel computational approach for prioritizing genes based on their relevance as drivers of disease progression and observed drug response. The system models a weighted combination of these features to learn each gene’s MERGE score, which indicates its potential reliability as a biomarker for drug sensitivity. Celik trained MERGE using publicly available AML data and gene annotation databases, along with gene expression data from 30 patients diagnosed with AML and their in vitro sensitivity data for 160 approved or experimental chemotherapy drugs. She then tested its performance against that of four existing methods for predicting patient drug response: correlation-based methods, ElasticNet, multi-task learning, and Bayesian multi-task multiple kernel learning (MKL).
Not only did MERGE outperform the current state of the art in its ability to accurately identify reliable biomarkers for drug response, but it discovered new gene-drug associations that those other methods failed to identify; these novel associations were validated in a biological laboratory. These findings are clinically important because they involve the drugs included in nearly all upfront AML treatment regimens today — mitoxantrone and etoposide.
“We ranked the top eight genes that were likely to be biologically significant for leukemia in several major drug classes,” explained Celik. “For five of those genes, MERGE was the only system capable of identifying their role in predicting treatment response and patient sensitivity to certain drugs — a significant improvement over existing approaches.”
One of those genes is the SMARCA4 gene expression, which the researchers determined and experimentally validated to be a sensitivity marker for a class of chemotherapy drugs known as topoisomerase II inhibitors. The MERGE analysis revealed that cell lines genetically engineered to show high SMARCA4 expression exhibit dramatically higher sensitivity to such drugs — making them excellent candidates for treating AML patients with high SMARCA4 expression.
“Drug development is an expensive and challenging process, and cancers that appear pathologically similar can respond to the same drug regimen in different ways,” noted Lee. “There are more than 1,200 potential cancer medicines in development in the United States alone. We need better methods for matching patients to the most effective treatment, and that has been our goal in developing MERGE.”
In addition to Lee, Celik, Blau, and Becker, contributors to the project include former Lee Lab postdoc Benjamin A. Logsdon of Sage Bionetworks; Allen School Ph.D. student Scott Lundberg; former Allen School Ph.D. student Javad Hosseini; Timothy Martins of the Quellos High Throughput Screening Core at UW’s Institute for Stem Cell & Regenerative Medicine; Vivian Oehler and Elihu Estey of Fred Hutchinson Cancer Research Center and UW Medicine; and Chris Miller, Sylvia Chien, Jin Dai, and Akanksha Saxena of UW Medicine. This work was supported by the National Institutes of Health, National Science Foundation, American Cancer Society, Life Sciences Discovery Fund, and philanthropic funding from Norman Metcalfe.
Read the full paper here, and visit the MERGE website here. Read a related UW Medicine story here and The Huddle article here.