Researchers in the Molecular Information Systems Lab (MISL) have taken another step forward in their quest to develop a next-generation data storage system with the introduction of new mechanisms for content-based similarity search of digital data stored in synthetic DNA. The team, which includes researchers from the University of Washington and Microsoft, took home the Best Student Paper Award in recognition of its work from the 24th International Conference on DNA Computing and Molecular Programming (DNA 24) in October.
The winning paper describes an end-to-end DNA-based architecture for content-based similarity search of stored media — in this case, image files. The MISL team’s contribution includes a novel neural-network-based sequence encoder trained on more than 30,000 images from the Caltech256 image dataset, and a laboratory experiment demonstrating the technique on a set of 100 test images.
Instead of encoding and storing the complete image files, the researchers concentrated on building a database for storing and retrieving their associated metadata. Each image’s encoded DNA strand includes a “feature sequence” representing its semantic features, as well as an “ID sequence” pointing to the location of the complete file in another database.
By adapting a technique from the machine learning community called “semantic hashing” to work with DNA sequences, the team designed an encoder to output feature sequences that react more strongly with the feature sequences of similar images. This enables a molecular “fishing hook”: when a molecule representing a query image is added to the database, similar images react with and stick to the query. The resulting query-target pairs can then be extracted using standard laboratory techniques like magnetic bead filtration.
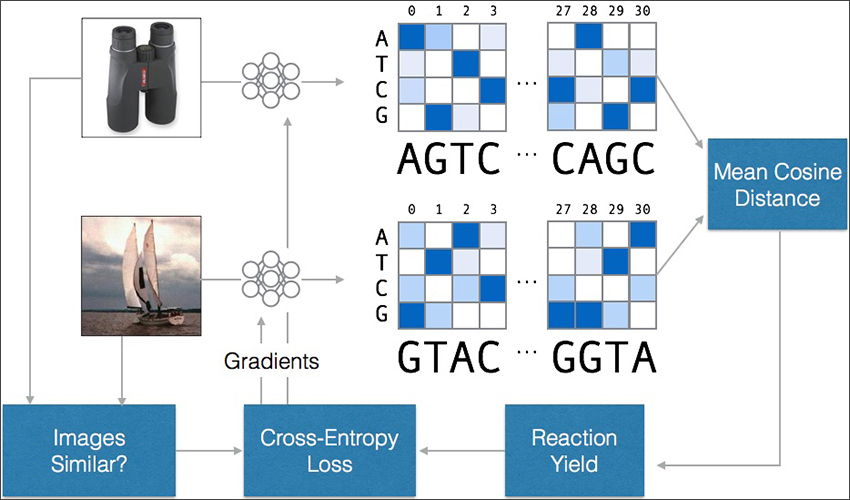
The training methodology used for the sequence encoder. A neural network translates images into DNA sequences, which are compared for similarity. If the sequences are similar but the images are not – or vice versa – the neural network is updated to increase its accuracy.
According to Allen School Ph.D. student and lead author Callista Bee, this type of efficient, content-based search mechanism will be key to unlocking DNA’s potential as the digital storage medium of the future.
“We’re approaching a time when zettabytes of data will be produced each year — a daunting challenge that requires us to think beyond the current state of the art,” Bee explained. “Our approach takes advantage of DNA’s near-data processing capabilities while borrowing from the latest machine learning techniques to present one possible solution for the indexing and retrieval of content-rich media.”
Bee’s co-authors on the work include Microsoft researcher Yuan-Jyue Chen, MISL members David Ward and Xiaomeng “Aaron” Liu, Allen School and Electrical & Computer Engineering professor Georg Seelig, Allen School professor Luis Ceze, and Microsoft senior researcher and Allen School affiliate professor Karin Strauss.
The team validated its design in wet-lab experiments using 100 target images and 10 query images, with 10 similar images for each query image included in the target set. The results, Bee said, were “moderately successful,” with visually similar files accounting for 30% of the sequencing reads for each query, despite comprising only 10% of the total database.
The researchers believe their approach can be generalized to databases containing any type of media. While it would be a challenge to scale such a system to larger and more complex datasets, the project opens up a promising new avenue of exploration around DNA-based data processing and content-based search.
Read the research paper here and learn more about MISL’s work here.
Congratulations to the entire team!