Allen School professors Tim Althoff and Carlos Guestrin were recognized by the Association for Computing Machinery’s Special Interest Group on Knowledge Discovery and Data Mining (SIGKDD) this week for seminal research contributions made roughly a dozen years apart.
At the KDD 2019 conference held in Anchorage, Alaska, Althoff received the 2019 SIGKDD Doctoral Dissertation Award for his paper “Data Science for Human Well-being” that presented new techniques for turning data generated by mobile and wearable devices into actionable insights to benefit individuals and society. And highlighting an example of enduring impact, SIGKDD recognized Guestrin and his co-authors with the 2019 Test of Time Award for their paper “Cost-effective Outbreak Detection in Networks” presenting a new methodology for detecting outbreaks in a network in the most efficient and effective manner.
Althoff, who earned his Ph.D. from Stanford University in 2018, devoted his graduate research to exploring how the multitude of data points about people’s offline behavior captured by mobile and wearable technologies can be used to improve physical and mental health and well-being. He and his collaborators developed a series of novel computational methods that leverage the proliferation of personal devices — and the digital traces of billions of human actions they capture — and techniques from data mining, social network analysis, and natural language processing to address significant health-related issues. For example, Althoff and his colleagues examined smartphone accelerometer data for more than 717,000 people across 111 countries — a planetary-scale analysis covering 68 million days of physical activity. The study uncovered a previously unknown health inequality about physical activity, with significantly reduced activity levels among the female portion of some populations. The researchers coined the term “activity inequality” to describe this difference in physical activity within countries and found that it serves as a better predictor of obesity prevalence than average activity volume. Their results also showed that features of the built environment, such as urban walkability, play an important role in reducing activity inequality and the associated gender gap.
Althoff was also one of the lead researchers behind the largest study to date to objectively measure the impact of sleep on performance in the wild by correlating data on 3 million nights of sleep measured by wearable devices with 75 million performance tasks in the form of search-engine interactions. These interactions, including keystroke speed and clicks, enabled the researchers to analyze how cognitive performance varies throughout the day tied to circadian rhythms, whether a subject is a “morning person” or a “night owl,” and the duration and timing of prior sleep. The team also developed a statistical model for determining the impact of insufficient sleep that found two consecutive nights of fewer than six hours of sleep leads to performance impairments lasting six days.
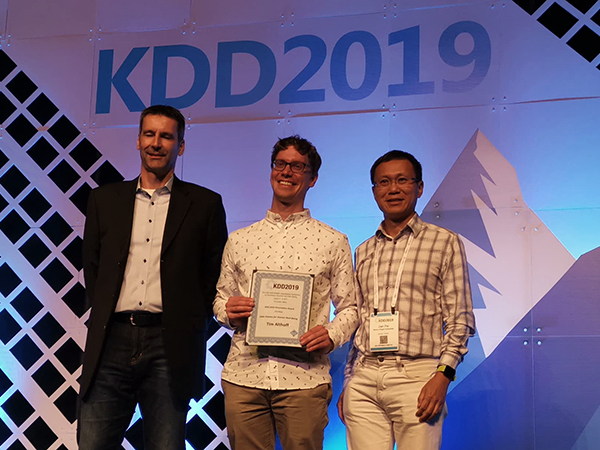
Althoff and his colleagues later developed a statistical tool, Time-varying, Interdependent, and Periodic Action Sequences (TIPAS), that models the complex dependencies and periodic recurrence of human behaviors associated with essential activities such as eating, exercise, and sleep. Testing their approach on 12 million actions taken by 20,000 users over the course of 17 months, Althoff’s team demonstrated that the model could be used to accurately predict future actions to enable targeted health interventions and app personalization.
In another example of how data analysis can yield actionable insights, Althoff and his colleagues also embarked on the largest-ever quantitative study of mental health counseling conducted via text messaging in an effort to identify the characteristics of successful counseling conversations. Applying techniques from natural language processing such as sequence-based conversation models, language model comparisons, message clustering, and word frequency analyses, the researchers sought to identify the conversational strategies associated with positive outcomes. They discovered a set of factors that were common among the most successful counselor-client interactions, including a counselor’s adaptability to the direction of the conversation; the extent to which they tailored their responses to the individual while using creative and personalized, rather than generic, language; and the ability to focus quickly on the core issue in order to move toward collaboratively solving the problem while facilitating a positive perspective change on the part of their client. The project represented the first time that researchers had connected large-scale data with labeled conversation outcomes to reveal the most effective conversational strategies in mental health counseling.
Guestrin and his then-colleagues at Carnegie Mellon University originally presented their paper on network outbreak detection at KDD 2007. Their work addressed the problem of how to optimize the placement of sensors or nodes to facilitate rapid detection of an outbreak or “information cascade” that initiates from a single node and spreads across the network — a question of both theoretical and practical significance. A network, in this case, could be physical, as in a water distribution system, or virtual, as in a social network or the blogosphere; an outbreak might consist of the spread of a physical contaminant within the system, or the viral spread of information online. The team presented a new algorithm, Cost-Effective Lazy Forward selection (CELF), that determines the near-optimal placement of sensors to detect such outbreaks, whether physical or virtual, by exploiting the principle of submodularity — the quality of exhibiting diminishing returns.
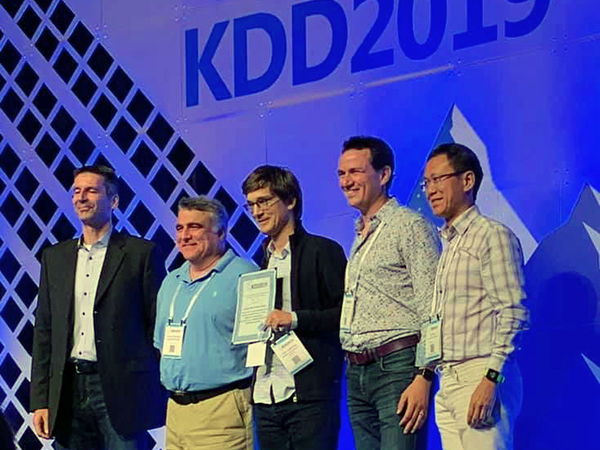
The central idea, drawing upon one of the paper’s real-world examples, is that the consumption of a blog post (placing of a sensor) yields more new information after having read only a few other blog posts than it does after having read many posts. The goal was to efficiently achieve a solution that minimizes cost — in the aforementioned instance, minimizing the time it takes to read multiple blog posts before detecting when a piece of information has gone viral. In another potential use case cited in the paper, the same algorithm could be used to speed the detection of a contaminant in the water supply before it reaches the broader population. Using this approach, the researchers were able to determine the near-optimal placement of sensors that enabled them to detect network outbreaks roughly 700 times faster than using a simple greedy algorithm. They also demonstrated that CELF could achieve speed-ups and storage savings of several orders of magnitude at scale. Last but not least, the team showed that the same methodology could be applied to the study of complex, application-specific questions around multicriteria tradeoff, cost-sensitivity analyses, and generalization behavior.
Guestrin’s co-authors on the paper include then-Ph.D. students Jure Leskovec, now a faculty member at Stanford University, and Andreas Krause, now a faculty member at ETH Zurich; CMU professors Christos Faloutsos and Jeanne VanBriesen; and Natalie Glance, former Senior Research Scientist at Nielsen BuzzMetrics and present Vice President of Engineering at DuoLingo.
Congratulations to Tim and to Carlos and his colleagues on their outstanding achievements!