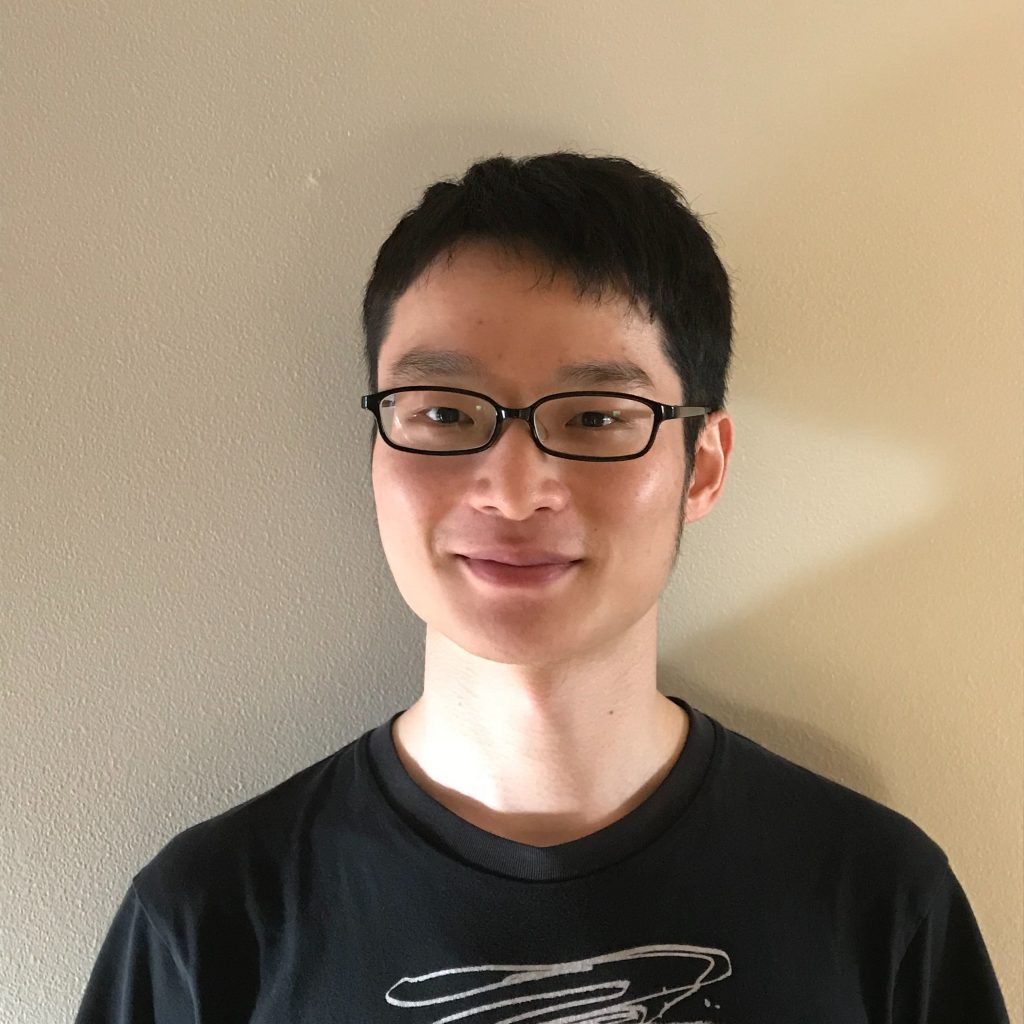
Jungo Kasai, a Ph.D. student working with Allen School professor Noah Smith on natural language processing (NLP), has been named a 2020 IBM Ph.D. Fellow. Kasai, who is one of only 24 students from a total of 140 universities around the world to be selected for a fellowship, was recognized in the “Artificial Intelligence/Cognitive Computing” category for his focus on the problem of cross-lingual transfer.
Deep learning has made incredible gains for NLP, but most of the research efforts have been focused on the English language. Real-world applications of NLP need to include a diverse set of languages. Kasai’s work questions whether or not we can exploit annotated data for “rich” languages like English to improve the accuracy of NLP components for other languages as well.
“My fundamental hypothesis is that different natural languages manifest similar characteristics which can be exploited by deep learning models and their distributed representations,” said Kasai. “I want to provide further support for this hypothesis by improving representation learning for diverse languages and ultimately to make contributions toward massively multilingual processing in the real world.”
So far, his research — which he is pursuing in collaboration with Allen School Ph.D. student Phoebe Mulcaire — is proving that hypothesis to be true. The team has worked to develop methods to produce multilingual representations from a large amount of monolingual data without extra annotation. They then created a multi-language program that models probability distribution over diverse languages and uses the distributed representations for any downstream task.
Kasai and Mulcaire published two papers last year showing that artificial intelligence models can be effectively expanded to many languages with little or even no labeled training data. The first paper, which the team presented at the 2019 conference of the North American Chapter of the Association for Computational Linguistics (NAACL), described Rosita. Rosita is a method for producing multilingual contextual word representations by training a single language model on text from multiple languages, such as English and Arabic or English and Chinese. The results showed the benefits of polyglot learning, in which representations are shared across multiple languages.
The second paper, presented at the 2019 conference of the ACL Special Interest Group on Natural Language Learning (CoNLL), takes their work even further, showing that the models can translate languages with complex language structures to those with more simplistic structures — or even none at all.
“Jungo and Phoebe’s paper delves into parsing and shows substantial gains in truly low-resource scenarios, including zero-shot parsing — i.e., learn only from English or another helper language — test it on sentences in a language with no training treebank at all,” said Smith. “The team’s work goes well beyond most publications in our field these days, which reveal new state-of-the-art scores on established benchmark tasks, and introduced an innovative technique for probing why the model works. I’m very proud of this work and Jungo’s contributions, which I believe will generate a lot of excitement and follow-on by others.”
Kasai is looking forward to meeting the other Ph.D. fellows and working with IBM.
“I believe working with industry is a great way to see my Ph.D. research on multilingual NLP and machine translation from different perspectives and to put research into practice,” he said.
Congratulations Jungo — and thanks to IBM for generously supporting student research!