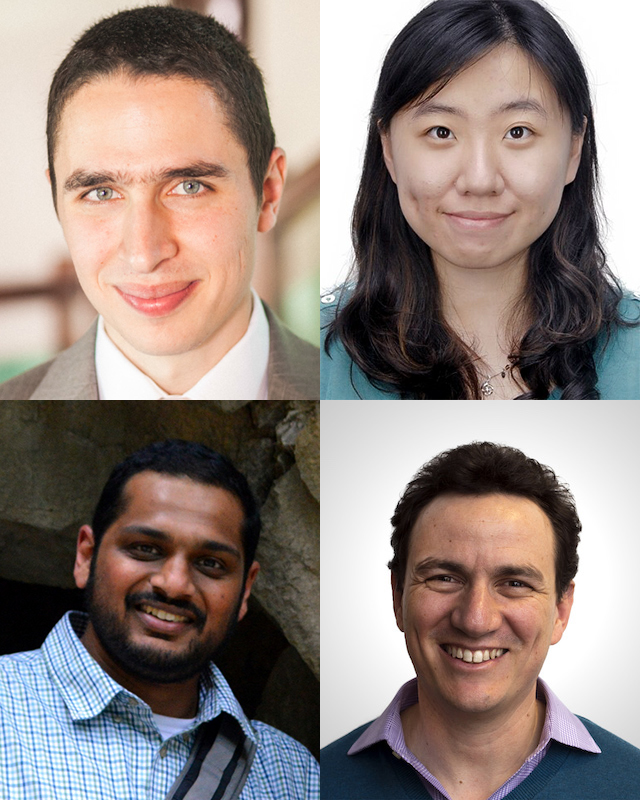
A team of researchers that includes Allen School professor Carlos Guestrin, Ph.D. student Tongshuang Wu and alumnus and affiliate professor Marco Tulio Ribeiro (Ph.D. ’18) of Microsoft captured the Best Paper Award at the 58th annual meeting of the Association for Computational Linguistics (ACL 2020). In the winning paper, “Beyond Accuracy: Behavioral Testing of NLP Models with CheckList,” they and former Allen School postdoc Sameer Singh, now a professor at the University of California, Irvine, introduce a methodology for comprehensively testing models for any natural language processing (NLP) task.
“In recent years, many machine learning models have achieved really high accuracy scores on a number of natural language processing tasks, often coming very close to or outperforming human accuracy,” Guestrin said. “However, when these models are evaluated carefully, they show critical failures and a basic lack of understanding of the tasks, indicating that these NLP tasks are far from solved.”
Much of lead author Ribeiro’s prior work is on analysis and understanding of machine learning and NLP models. As the driving force of the project, he began testing and found critical failures in several state-of-the-art models used for research and commercial purposes — despite the fact that these models out-performed humans on standard benchmarks. To remedy this problem, Ribeiro and his colleagues created a process and a tool called CheckList, which provides the framework and tooling necessary to enable developers to break their tasks into different “capabilities” and then write tests for these using a variety of test types.
People in several industries found new and actionable bugs to fix by using CheckList, even in systems that had been extensively tested in the past. In fact, NLP practitioners found three times more bugs by using CheckList than compared to existing techniques.
“I did a case study with a team at Microsoft and they were very happy to discover a lot of new bugs with CheckList”,” Ribeiro said. “We also tested models from Google and Amazon and we’ve received positive feedback from researchers in both companies.”
Including CheckList, University of Washington researchers earned three out of the five recognized paper awards at ACL this year. The second of these, a Best Paper Honorable Mention, went to a group of researchers that included professor Noah Smith, Ph.D. student Suchin Gururangan, and postdocs Ana Marasović and Swabha Swayamdipta for their work on “Don’t Stop Pretraining: Adapt Language Models to Domains and Tasks.” The team — which also included applied research scientists Kyle Lo and Iz Beltagy of AI2 and Northwestern University professor Doug Downey — explored whether they could apply a multi-phased, adaptive approach to pretraining to improve the performance of language models that are pretrained on text from a wide variety of sources, from encyclopedias and news articles, to literary works and web content. Using a method they referred to as domain-adaptive pretraining (DAPT), the researchers showed how tailoring a model to the domain of a specific task can yield significant gains in performance. Further, the team found that by adapting the model to a task’s unlabeled data — an approach known as task-adaptive pretraining (TAPT) — they could boost the model’s performance with or without the use of DAPT.
In addition, Allen School adjunct professor Emily Bender of the UW Department of Linguistics and Saarland University professor Alexander Koller were awarded Best Theme Paper for “Climbing towards NLU: On Meaning, Form, and Understanding in the Age of Data,” in which they draw a distinction between form and meaning in assessing the field’s progress toward natural language understanding. While recognizing the success of large neural models like BERT on many NLP tasks, the authors assert that such systems cannot capture meaning from linguistic form alone. Instead, Bender and Koller argue, these models have been shown to learn some reflection of meaning into the linguistic form that has proven useful in various applications. With that in mind, the authors offer some thoughts on how members of their field can maintain a healthy — but not overhyped — optimism with respect to communicating about their work with language models and the overall progress of the field.
Congratulations to all on an outstanding showing at this year’s conference!