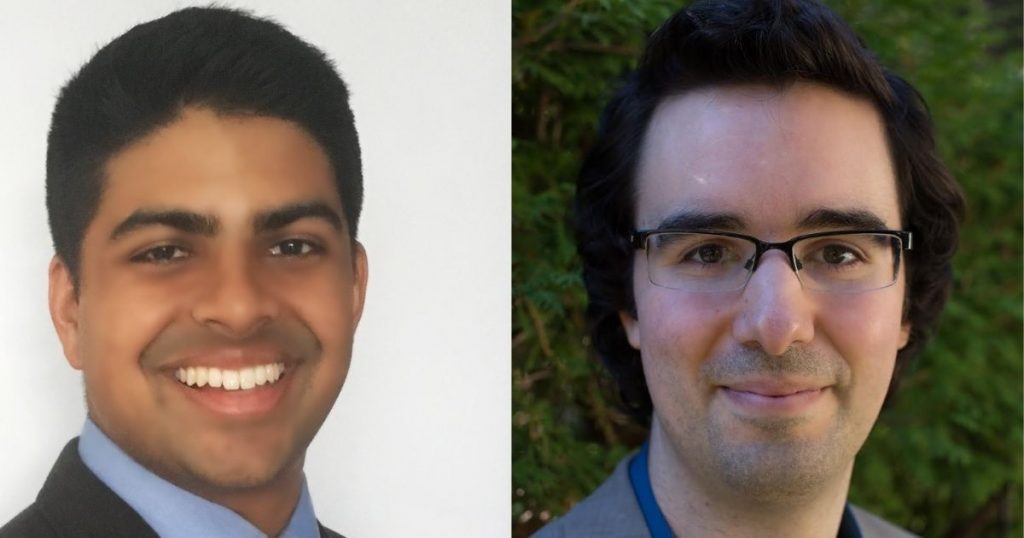
Allen School Ph.D. students Vivek Jayaram and John Thickstun have been named 2020 Qualcomm Innovation Fellows for their work in signal processing, computer vision and machine learning using the latest in generative modeling to improve source separation. In their paper, “Source Separation with Deep Generative Priors,” published at the 2020 International Conference on Machine Learning, the team addresses perceptible artifacts that are often found in source separation algorithms. Jayaram and Thickstun are one of only 13 teams to receive a fellowship out of more than 45 finalists across North America.
Thickstun and Jayarum have been working with their advisors, Allen School professors Sham Kakade, Steve Seitz, and Ira Kemelmacher-Shlizerman and adjunct faculty member Zaid Harchaoui, a professor in the UW Department of Statistics, on this research. Potential applications include separating reflections from an image, voices of multiple speakers or instruments from an audio recording, brain signals in an EEG and telecommunication technologies from Code Division Multiple Access (CDMA). The team’s work introduces a new algorithmic idea for solving source separation problems using a Bayesian approach.
“In contrast to source separation models, modern generative models are largely free of artifacts,” said Thickstun. “Generative models continue to improve and one goal of our proposal is to find a way to use the latest advances in generative modeling to improve source separation results.”
Employing a cutting-edge generative model is a powerful tool for source separation and can be applied to different data domains. Using the Bayesian approach and Langevin dynamics, Thickstun and Jayaram can decouple the source separation problem from the generative model, achieving a state-of-the-art performance for separation of low resolution images.
By combining images and using the algorithm to then separate each of them, the team was able to illustrate how their theory works.
“Our algorithm works on mixtures of any number of components without retraining,” Jayaram said. “The only training is a generative model of the original images themselves; we never train it on mixtures of a fixed number of sources.”
Audio separation proved to be more challenging, but the two implemented Stochastic gradient Langevin dynamics to speed up the process and make it more practical. Their approach can be modified to tackle many different strains of optimization problems by modifying the reconstruction objective.
“John and Vivek’s work takes a fundamentally new and promising approach that leverages the power of deep networks to help separate out signals,” Kakade said. “The reason this approach is so exciting is that deep learning methods have already demonstrated remarkable abilities to model distributions, and their work looks to harness these models for the classical signal processing problem of source separation.”
Jayaram and Thickstun have each published additional papers in advancing source separation at the 2020 IEEE Conference on Computer Vision and Pattern Recognition (Jayaram), which focuses on background matting in images, and the 2019 International Society for Music Information Retrieval (Thickstun), which investigates end-to-end learnable models for attributing composers to musical scores.
Since 2009, the Qualcomm Innovation Fellowship program has recognized and supported innovative graduate students across a broad range of technical research areas. Previous Allen School recipients include Vincent Lee and Max Willsey (2017), Hanchuan Li and Alex Mariakakis (2016), Carlo del Mundo and Vincent Lee (2015), Vincent Liu and Vamsi Talla (2014) and Adrian Sampson and Theirry Moreau (2013). Learn more about the 2020 Qualcomm Fellows here.
Congratulations, Vivek and John!