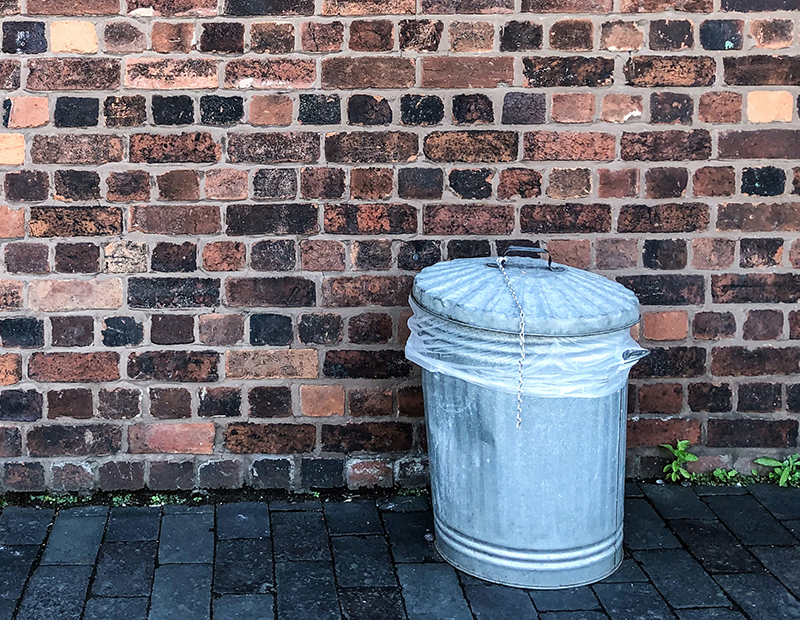
In the spring of 2016, social media users turned a friendly online chatbot named Tay — a seemingly innocuous experiment by Microsoft in which the company invited the public to engage with its work in conversational learning — into a racist, misogynistic potty mouth that the company was compelled to take offline the very same day that it launched. Two years later, Google released its Smart Compose tool for Gmail, a feature designed to make drafting emails more efficient by suggesting how to complete partially typed sentences that also had an unfortunate tendency to suggest a bias towards men — leading the company to eschew the use of gendered pronouns altogether.
These and other examples serve as a stark illustration of that old computing adage “garbage in, garbage out,” acknowledging that a program’s outputs can only be as good as its inputs. Now, thanks to a team of researchers at the Allen School and Allen Institute for Artificial Intelligence (AI2), there is a methodology for examining just how trashy some of those inputs might be when it comes to pretrained neural language models — and how this causes the models themselves to degenerate into purveyors of toxic content.
The problem, as Allen School Master’s student Samuel Gehman (B.S., ‘19) explains, is that not all web text is created equal.
“The massive trove of text on the web is an efficient way to train a model to produce coherent, human-like text of its own. But as anyone who has spent time on Reddit or in the comments section of a news article can tell you, plenty of web content is inaccurate or downright offensive,” noted Gehman. “Unfortunately, this means that in addition to higher quality, more factually reliable data drawn from news sites and similar sources, these models also take their cues from low-quality or controversial sources. And that can lead them to churn out low-quality, controversial content.”
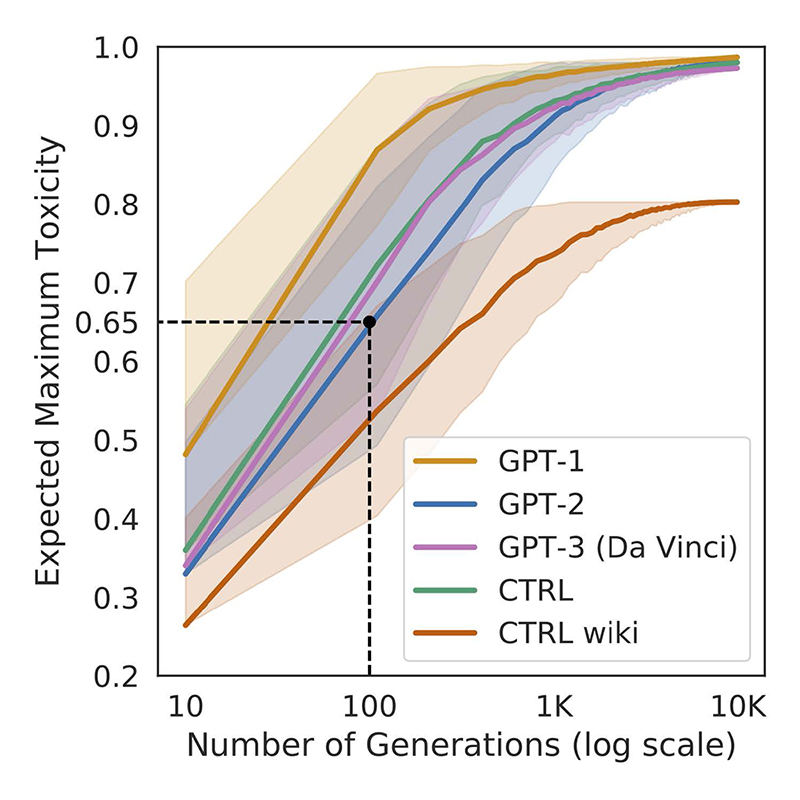
Gehman and the team set out to measure how easily popular neural language models such as GPT-1, GPT-2, and CTRL would begin to generate problematic outputs. The researchers evaluated the models using a testbed they created called RealToxicityPrompts, which contains 100,000 naturally occurring English-language prompts, i.e., sentence prefixes, that models have to finish. What they discovered was that all three were prone to toxic degeneration even with seemingly innocuous prompts; the models began generating toxic content within 100 generations, and exceeded expected maximum toxicity levels within 1,000 generations.
The team — which includes lead author Gehman, Ph.D. students Suchin Gururangan and Maarten Sap, and Allen School professors and AI2 researchers Yejin Choi and Noah Smith — published its findings in a paper due to appear at the next conference on Findings of Empirical Methods in Natural Language Processing (Findings of EMNLP 2021).
“We found that if just 4% of your training data is what we would call ‘highly toxic,’ that’s enough to make these models produce toxic content, and to do so rather quickly,” explained Gururangan. “Our research also indicates that existing techniques that could prevent such behavior are not effective enough to safely release these models into the wild.”
That approach, in fact, can backfire in unexpected ways, which brings us back around to Tay — or rather, Tay’s younger “sibling,” Zo. When Microsoft attempted to rectify the elder chatbot’s propensity for going on racist rants, it scrubbed Zo clean of any hint of political incorrectness. The result was a chatbot that refused to discuss any topic suggestive of religion or politics, such as the time a reporter simply mentioned that they live in Iraq and wear a hijab. When the conversation steered towards such topics, Zo’s response would become agitated; if pressed, the chatbot might terminate the conversation altogether.
As an alternative to making certain words or topics automatically off-limits — a straightforward solution but one that lacked nuance, as evidenced by Zo’s refusal to discuss subjects that her filters deemed controversial whether they were or not — Gururangan and his collaborators explored how the use of steering methods such as the fine-tuning of a model with the help of non-toxic data might alleviate the problem. They found that domain-adaptive pre-training (DAPT), vocabulary shifting, and PPLM decoding showed the most promise for reducing toxicity. But it turns out that even the most effective steering methods have their drawbacks: in addition to being computationally and data intensive, they could only reduce, not prevent, neural toxic degeneration of a tested model.
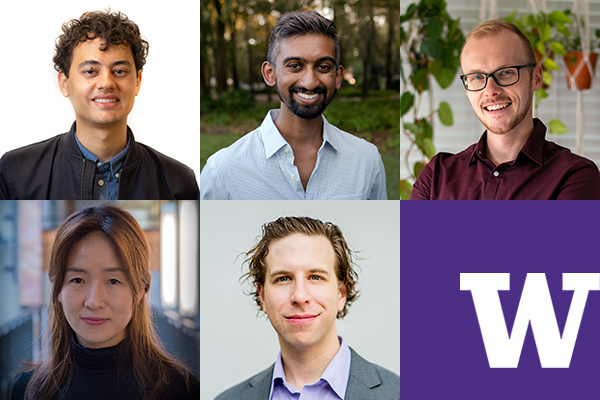
Having evaluated more conventional approaches and found them lacking, the team is encouraging an entirely new paradigm when it comes to pretraining modern NLP systems. The new framework calls for greater care in the selection of data sources and more transparency around said sources, including public release of original text, source URLs, and other information that would enable a more thorough analysis of these datasets. It also encourages researchers to incorporate value-sensitive or participatory design principles when crafting their models.
“While fine-tuning is preferable to the blunt-instrument approach of simply banning certain words, even the best steering methods can still go awry,” explained Sap. “No method is foolproof, and attempts to clean up a model can have had the unintended consequence of shutting down legitimate discourse or failing to consider language within relevant cultural contexts. We think the way forward is to ensure that these models are more transparent and human-centered, and also reflect what we refer to as algorithmic cultural competency.”
Learn more by visiting the RealToxicityPrompts project page here, and read the research paper here. Check out the AI2 blog post here, and a related Fortune article here.