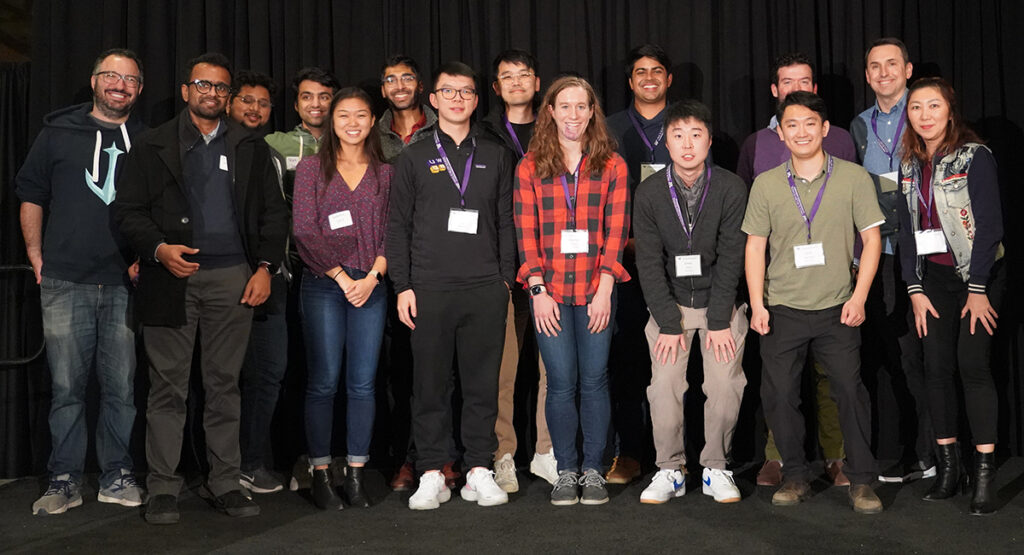
After a pandemic-enforced hiatus, last week the Allen School welcomed industry partners, alumni and friends to its 2022 Research Showcase this week to celebrate the groundbreaking work of its students and faculty. The typically annual event, which is hosted by the Industry Affiliates Program, welcomes industry partners and alumni to engage with the school’s research and learn more about how its members are advancing the field of computing. This year marked the first time the showcase has been held since 2019.
The agenda included a variety of technical sessions featuring the latest and greatest Allen School research. Topics ranged from computing for the environment and artificial intelligence for health, to robotics and computational biology. The day concluded with an open house and poster session that culminated in the announcement of the Madrona Prize and People’s Choice Awards.
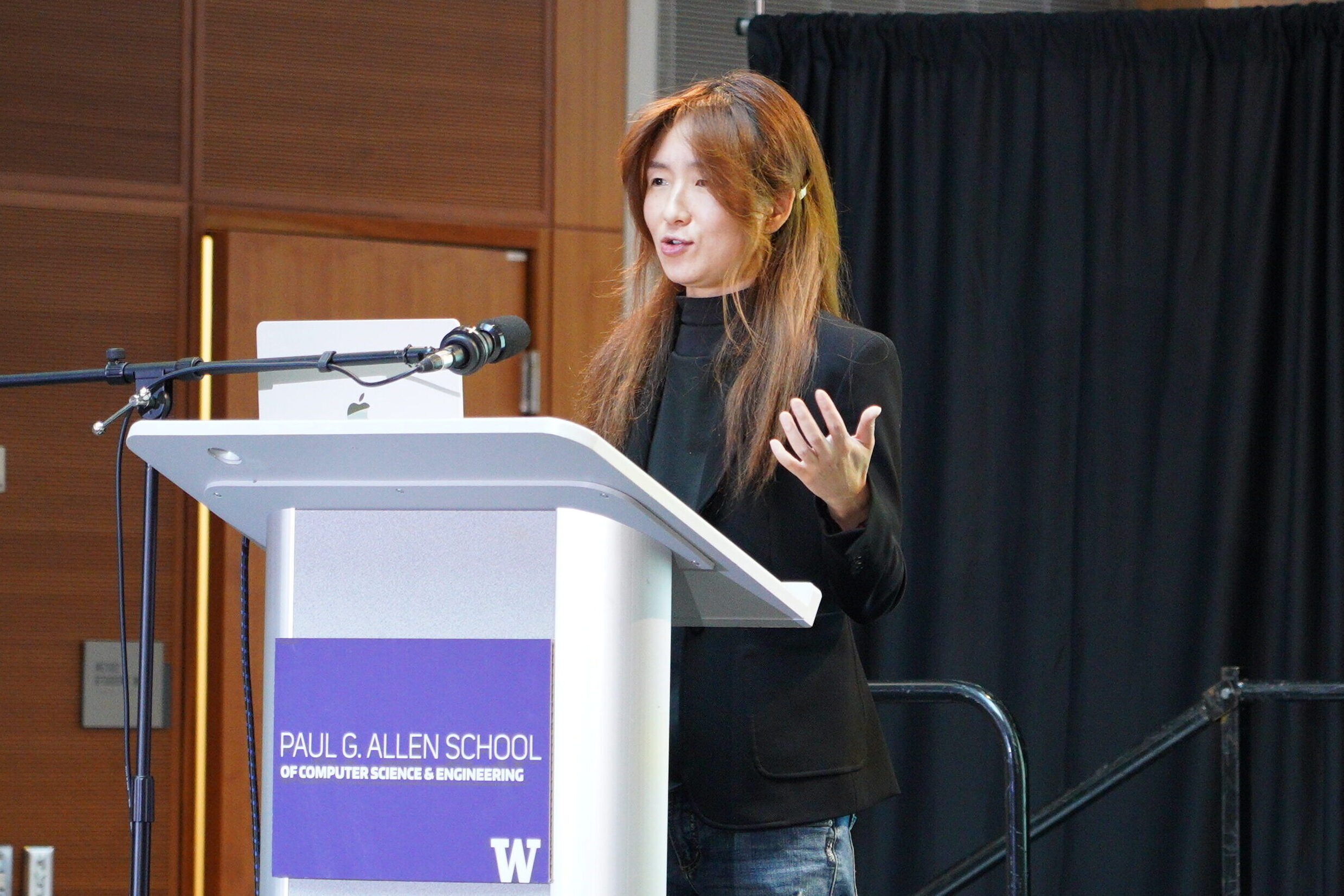
Allen School professor Yejin Choi, who was recently named a MacArthur Fellow for her work in natural language processing and commonsense AI, gave the keynote address titled “David vs. Goliath: The Art of Leaderboarding in the Era of Extreme Scale Neural Models.” During the talk, she highlighted the power of smaller neural models developed in academia and how they can have an edge over larger industry-scale models.
One of the approaches that gives them this edge, Choi explained, is Symbolic Knowledge Distillation, a new framework she and her colleagues proposed that distills knowledge symbolically as text besides just the neural model alone. Her work produced a machine-authored commonsense model that, for the first time, surpassed a human-authored model in all criteria, including scale, accuracy and diversity. Instead of humans directing the commonsense knowledge graph, Choi and her collaborators found that through their framework machines could write their own knowledge graph, teaching themselves to distill language, knowledge and reasoning.
Choi also explained how an unsupervised, inference-time reasoning algorithm, can match or surpass supervised approaches on hard-reasoning tasks or complex language generation tasks that require logical constraints. The algorithm, called NeuroLogic Decoding, illustrated the limits of larger-scale neural models while also demonstrating better performance on text-generation tasks.
While bullish on the potential of smaller, high-quality models, Choi acknowledged that scaling is a necessary condition for making progress in AI — necessary, but not sufficient.
“Scaling laws are real, and denial is futile,” said Choi, the Bret Helsel Professor in the Allen School and senior research manager at the Allen Institute for AI. “Especially when we think about hard problems in AI, we cannot just solve the hardest problems by scaling things up — analogous to how you cannot reach to the moon by making the tallest building in the world one inch taller at a time.”
In the evening, nearly 300 people came together in the Paul G. Allen Center to catch up after two years of pandemic-enforced absence and view the latest research from Allen School labs. More than 50 teams of student researchers shared their work with attendees, who were invited to vote for their favorite poster or demo as part of the People’s Choice Award.
Members of Madrona Venture Group, longtime friends and supporters of the Allen School, were on hand to present the Madrona Prize, which recognizes exciting projects with commercialization potential. Madrona partner Chris Picardo announced that members of the UW Database Group behind VOCAL — short for Video Organization and Interactive Compositional AnaLytics — captured top honors for two projects related to that work. VOCAL allows users to extract semantic content from large datasets while minimizing inefficiencies in data cleaning, compositional queries, exploration and organization.
“We’re delighted to be back and able to award the prize again and have an amazing poster session,” Picardo said. “We’re thrilled that we get to do this and get to see such amazing research.”
2022 Madrona Prize
Winner
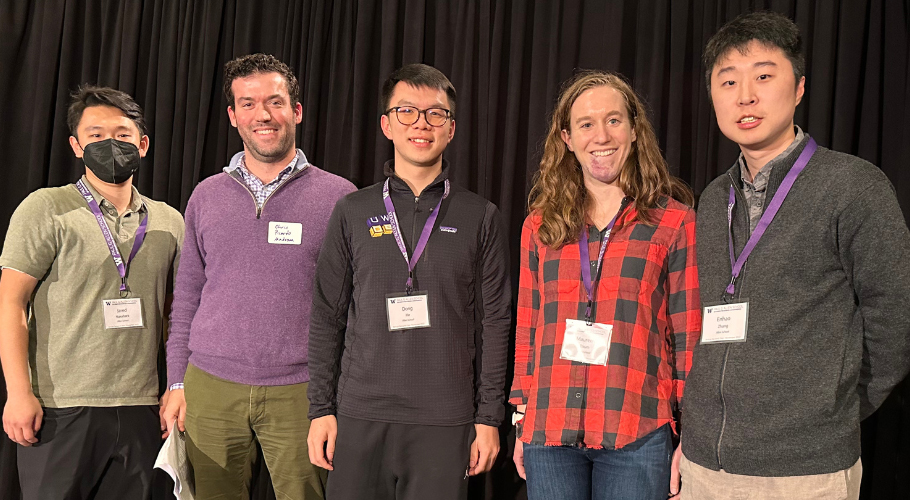
Video Organization and Exploration and Interactive Video Analytics for Compositional Queries: Ph.D. students Maureen Daum, Enhao Zhang and Dong He; Allen School director and professor Magdalena Balazinska; professor Ranjay Krishna; alum Brandon Haynes (Ph.D., ‘20), now a senior scientist at Microsoft
Runners up
Clearbuds: Wireless Binaural Earbuds for Learning-Based Speech Enhancement: Ph.D. students Ishan Chatterjee, Maruchi Kim and Vivek Jayaram; professors Shyam Gollakota, Ira Kemelmacher-Shlizerman, Shwetak Patel and Steven M. Seitz
Data Analysis Systems for Statistical Non-Experts: Ph.D. student Eunice Jun; professors Jeffrey Heer and René Just
Levity: Contactless Robotics and Automation for Synthetic Biology: Ph.D. student Jared Nakahara; professor Joshua R. Smith
2022 People’s Choice Awards
Distributing Trust and Establishing Transparency in Societal Scale Computing and Network Infrastructure: Ph.D. students Sudheesh Singanamalla, Matthew Johnson and Esther Han Beol Jang; master’s students Nick Durand and Abhishek Shah; postdoctoral researcher Spencer Sevilla; professors Richard Anderson and Kurtis Heimerl
Beyond WEIRDness of NLP: Ph.D. student Sebastin Santy; professors Katharina Reinecke and Yulia Tsvetkov; alum and former AI2 postdoc Maarten Sap (Ph.D., ‘21); psychology professor Andrew Meltzoff; research scientist Ronan Le Bras of the Allen Institute for AI; undergraduate alum Jenny Liang (B.S., ‘21), now a Ph.D. student at Carnegie Mellon University; research scientist Rodolfo Barragan of the UW Institute for Learning & Brain Sciences
Read GeekWire’s coverage of the awards here.
Thank you to our friends at Madrona and all of the members of the extended Allen School community who joined us in celebrating and supporting student innovation — it was wonderful to see you again!