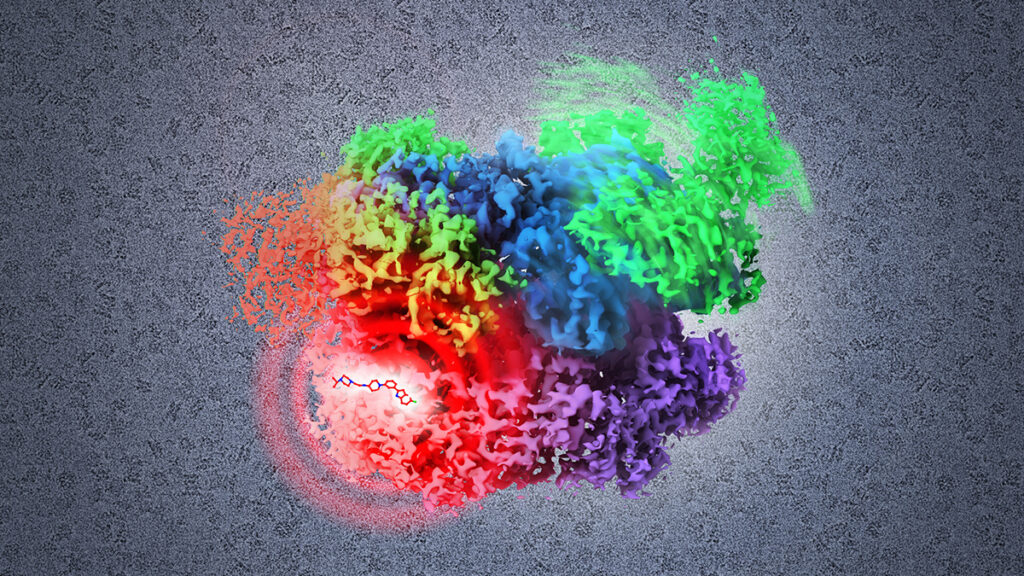
When the novel coronavirus SARS-Cov-2 began sweeping across the globe, scientists raced to figure out how the virus infected human cells so they could halt the spread.
What if scientist had been able to simply type a description of the virus and its spike protein into a search bar, and received information on the angiotensin-converting enzyme 2 — colloquially known as the ACE2 receptor, through which the virus infects human cells — in return? And what if, in addition to identifying the mechanism of infection for similar proteins, this same search also returned potential drug candidates that are known to inhibit their ability to bind to the ACE2 receptor?
Biomedical research has yielded troves of data on protein function, cell types, gene expression and drug formulas that hold tremendous promise for assisting scientists in responding to novel diseases as well as fighting old foes such as Alzheimer’s, cancer and Parkinson’s. Historically, their ability to explore these massive datasets has been hampered by an outmoded model that relied on painstakingly annotated data, unique to each dataset, that precludes more open-ended exploration.
But that may be about to change. In a recent paper published in Nature Communications, Allen School researchers and their collaborators at Microsoft and Stanford University unveiled BioTranslator, the first multilingual translation framework for biomedical research. BioTranslator — a portmanteau of “biological” and “translator” — is a state-of-the-art, zero-shot classification tool for retrieving non-text biological data using free-form text descriptions.
“BioTranslator serves as a bridge connecting the various datasets and the biological modalities they contain together,” explained lead author Hanwen Xu, a Ph.D. student in the Allen School. “If you think about how people who speak different languages communicate, they need to translate to a common language to talk to each other. We borrowed this idea to create our model that can ‘talk’ to different biological data and translate them into a common language — in this case, text.”
The ability to perform text-based search across multiple biological databases breaks from conventional approaches that rely on controlled vocabularies (CVs). As the name implies, CVs come with some constraints. Once the original dataset is created via the painstaking process of manual or automatic annotation according to a predefined set of terms, it is difficult to extend them to the analysis of new findings; meanwhile, the creation of new CVs is time consuming and requires extensive domain knowledge to compose the data descriptions.
BioTranslator frees scientists from this rigidity by enabling them to search and retrieve biological data with the ease of free-form text. Allen School professor Sheng Wang, senior author of the paper, likens the shift to when the act of finding information online progressed from combing through predefined directories to being able to enter a search term into open-ended search engines like Google and Bing.
“The old Yahoo! directories relied on these hierarchical categories like ‘education,’ ‘health,’ ‘entertainment’ and so on. That meant that If I wanted to find something online 20 years ago, I couldn’t just enter search terms for anything I wanted; I had to know where to look,” said Wang. “Google changed that by introducing the concept of an intermediate layer that enables me to enter free text in its search bar and retrieve any website that matches my text. BioTranslator acts as that intermediate layer, but instead of websites, it retrieves biological data.”
Wang and Xu previously explored text-based search of biological data by developing ProTranslator, a bilingual framework for translating text to protein function. While ProTranslator is limited to proteins, BioTranslator is domain-agnostic, meaning it can pull from multiple modalities in response to a text-based input — and, as with the switch from old-school directories to modern search engines, the person querying the data no longer has to know where to look.
BioTranslator does not merely perform similarity search on existing CVs using text-based semantics; instead, it translates the user-generated text description into a biological data instance, such as a protein sequence, and then searches for similar instances across biological datasets. The framework is based on large-scale pretrained language models that have been fine-tuned using biomedical ontologies from a variety of related domains. Unlike other language models that are having a moment — ChatGPT comes to mind — BioTranslator isn’t limited to searching text but rather can pull from various data structures, including sequences, vectors and graphs. And because it’s bidirectional, BioTranslator not only can take text as input, but also generate text as output.
“Once BioTranslator converts the biological data to text, people can then plug that description into ChatGPT or a general search engine to find more information on the topic,” Xu noted.
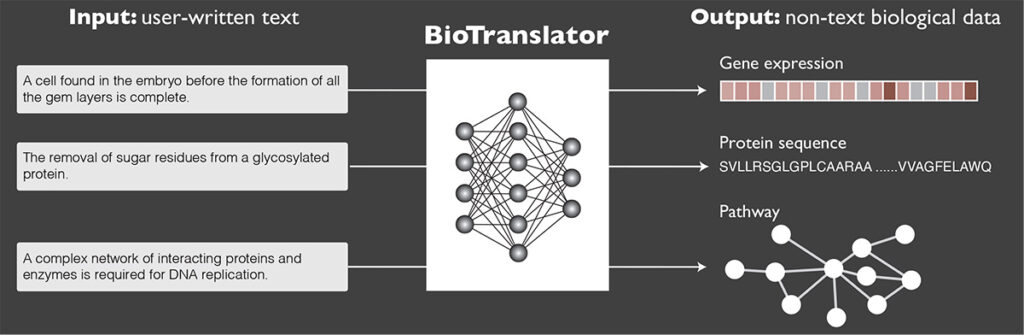
Xu and his colleagues developed BioTranslator using an unsupervised learning approach. Part of what makes BioTranslator unique is its ability to make predictions across multiple biological modalities without the benefit of paired data.
“We assessed BioTranslator’s performance on a selection of prediction tasks, spanning drug-target interaction, phenotype-gene association and phenotype-pathway association,” explained co-author and Allen School Ph.D. student Addie Woicik. “BioTranslator was able to predict the target gene for a drug using only the biological features of the drugs and phenotypes — no corresponding text descriptions — and without access to paired data between two of the non-text modalities. This sets it apart from supervised approaches like multiclass classification and logistic regression, which require paired data in training.”
BioTranslator outperformed both of those approaches in two out of the four tasks, and was better than the supervised approach that doesn’t use class features in the remaining two. In the team’s experiments, BioTranslator also successfully classified novel cell types and identified marker genes that were omitted from the training data. This indicates that BioTranslator can not only draw information from new or expanded datasets — no additional annotation or training required — but also contribute to the expansion of those datasets.
“The number of potential text and biological data pairings is approaching one million and counting,” Wang said. “BioTranslator has the potential to enhance scientists’ ability to respond quickly to the next novel virus, pinpoint the genetic markers for diseases, and identify new drug candidates for treating those diseases.”
Other co-authors on the paper are Allen School alum Hoifung Poon (Ph.D., ‘11), general manager at Microsoft Health Futures, and Dr. Russ Altman, the Kenneth Fong Professor of Bioengineering, Genetics, Medicine and Biomedical Data Science, with a courtesy appointment in Computer Science, at Stanford University. Next steps for the team include expanding the model beyond expertly written descriptions to accommodate more plain language and noisy text.
Read the Nature Communications paper here, and access the BioTranslator code package here.