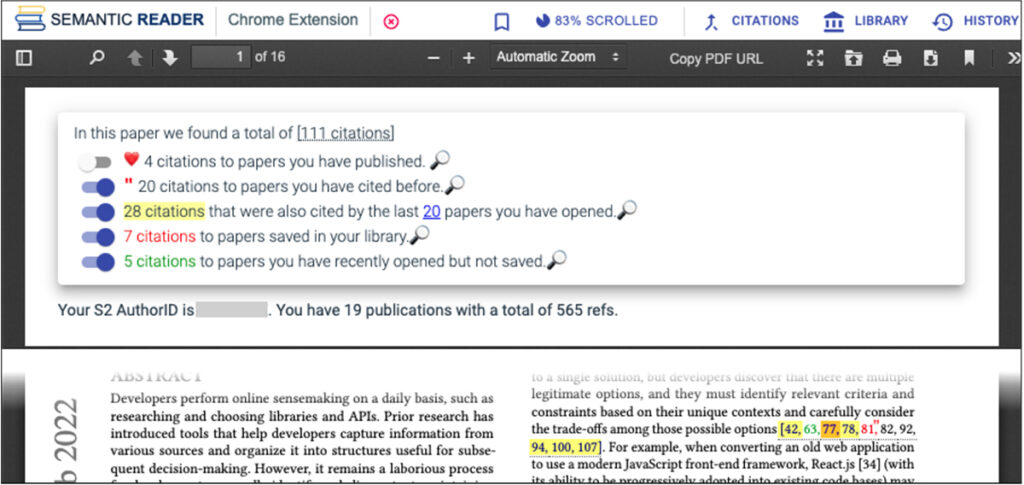
The review of existing literature is an essential part of scientific research — and citations play a key role by enabling researchers to trace the origins of ideas, put the latest progress into context and identify potential directions for future research. In the course of their review, a researcher may encounter dozens, or even hundreds, of inline citations that may or may not be linked to papers that are directly relevant to their work. While there are tools that can predict how a cited paper contributed or weigh its influence on a particular work, those are one-size-fits-all approaches that do not reflect a reviewer’s personal interests and goals.
A team of researchers from the Allen School, Allen Institute for AI, and the University of Pennsylvania envisioned a more personalized experience. The resulting paper, “CiteSee: Augmenting Citations in Scientific Papers with Persistent and Personalized Historical Context,” recently earned a Best Paper Award at the Association for Computing Machinery Conference on Human Factors in Computing Systems (CHI 2023).
CiteSee assists researchers with scientific literature reviews by creating personalized visual cues to contextualize previously encountered citations. These cues make it easier to identify potentially relevant papers which they have yet to consider — and keep tabs on those they encountered previously — based on their reading and saving history.
To inform their design of the tool, the team conducted exploratory interviews to understand how researchers make sense of inline citations as they read as well as what limitations and needs come up when completing literature reviews.
”We discovered that researchers had trouble determining which citations were important,” explained co-author and Allen School professor Amy Zhang, director of the Social Futures Lab. “They also wanted to keep better track of the context around their saved citations, especially when those papers appeared as citations in multiple publications.”
Based on their findings, Zhang and her colleagues built three key features into CiteSee to personalize the reader’s experience. These included the means to augment known paper citations, discover unknown paper citations, and assist users in keeping track of and triaging how they had previously interacted with papers to make better sense of inline citations as they read. CiteSee applies visual cues to indicate to readers whether a particular citation is one they have previously encountered, such as a previously visited paper, a saved paper, a paper that the reader has cited in the past or the reader’s own paper.
“A user can adjust the time frame around papers they have read to fine-tune CiteSee’s visual augmentation of inline citations,” noted Zhang. “They can also easily reference the citing sentence for that same citation across multiple papers, enabling them to truly customize the literature review experience.”
The research team assessed how useful CiteSee would be in practice through both a controlled laboratory study and a field deployment. In the lab study, the researchers aimed to validate CiteSee’s ability to identify relevant prior work during the literature review process. They found that leveraging personal reading history had a significant impact on CiteSee’s ability to identify relevant citations for the users.
The goal of the field deployment was to observe how CiteSee would perform in a real-world literature review setting. Based on their observations and subsequent interviews, the team found that participants actively engaged with augmented inline citations. They also found that having the ability to view the citations in context aided the participants to make connections across papers. In addition, when participants opened reencountered citations, they were three times more likely to discover relevant prior work than through other methods. Overall, participants found that CiteSee enabled them to keep better track of reencountered citations, process current papers for relevant citations, recall previously read papers and understand relationships through sensemaking across multiple papers. Two-thirds of the participants continued to engage with CiteSee following the research period.
Beyond this, the team identified a couple of features to potentially incorporate into CiteSee in the future, such as providing the means to specify the current literature review context and also to differentiate between multiple simultaneous literature searches.
In addition to Zhang, co-authors of the paper include Allen School professor emeritus Daniel Weld, general manager and chief scientist for Semantic Scholar at AI2; Allen School alumni Jonathan Bragg (Ph.D., ‘18) and Doug Downey (Ph.D., ’08), who are both senior research scientists at AI2; lead author Joseph Chee Chang, senior research scientist at AI2; AI2 scientist Kyle Lo; and University of Pennsylvania professor Andrew Head.
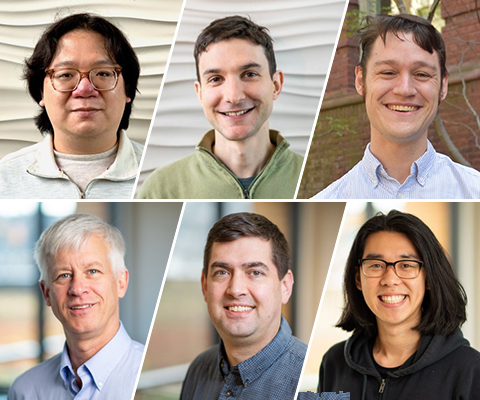
Three additional papers by Allen School researchers received honorable mentions at this year’s CHI conference.
Amanda Baughan, a fifth-year Ph.D. student advised by Allen School adjunct professor Alexis Hiniker, a faculty member in the Information School, was lead author of “A Mixed-Methods Approach to Understanding User Trust after Voice Assistant Failures.” Baughan and her co-authors at Google Research developed a crowdsourced dataset of voice assistant failures to analyze how failures affect users’ trust in their voice assistants and approaches for regaining trust.
Allen School professor Katharina Reinecke, director of the Wildlab, co-authored “Why, When, and for Whom: Considerations for Collecting and Reporting Race and Ethnicity Data in HCI.” In an effort to improve the field’s engagement of diverse participants and generate safe, inclusive and equitable technology, Reinecke and her collaborators at Stanford University, University of Texas at Austin and Northeastern University investigated current approaches to the collection of race and ethnicity data and offered a set of principles for HCI researchers to consider concerning when and how to include such data in future.
Allen School professor Leilani Battle (B.S., ’11) co-director of the Interactive Data Lab, Allen School alumna and lead author Deepthi Raghunandan (M.S., ’16) and colleagues at the University of Maryland explored the iterative process of sensemaking in their paper, “Code Code Evolution: Understanding How People Change Data Science Notebooks Over Time.” The team analyzed over 2500 Jupyter notebooks from Github to determine how notebook authors participate in sensemaking activities such as branching analysis, annotation and documentation, and offered recommendations for extensions to notebook environments to better support such activities.
For more information on CiteSee, read a related AI2 blog post here. In addition to Best Paper honors, multiple UW and Allen School-affiliated researchers were individually recognized with SIGCHI Awards at this year’s conference. These include Nicola Dell (Ph.D., ‘15), now faculty at Cornell University, and Outstanding Dissertation Award recipient Dhruv Jain (Ph.D., ‘21), now a faculty member at the University of Michigan. For a comprehensive overview of all UW authors’ contributions at CHI 2023, read the DUB roundup here.