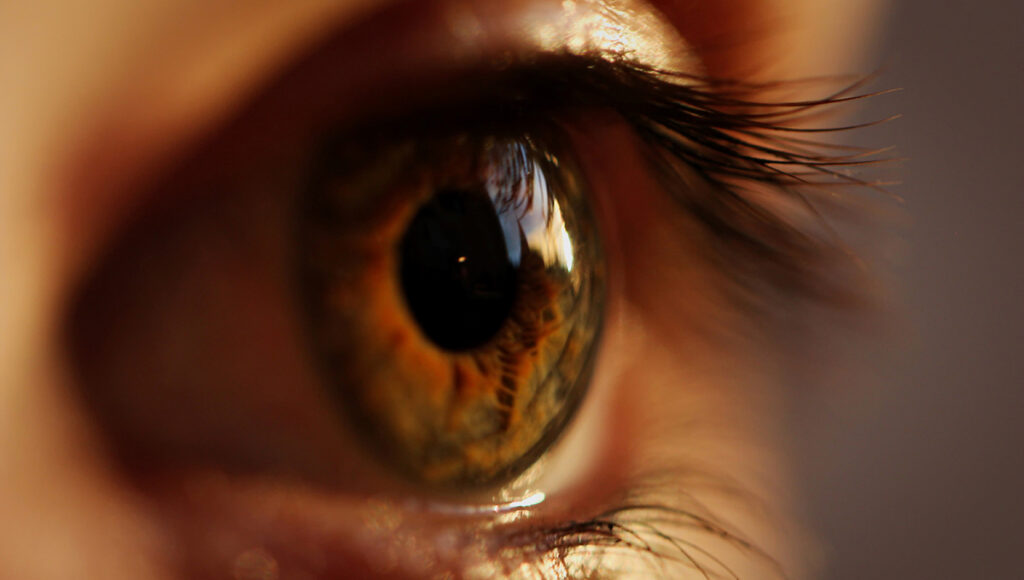
There is an old saying that perception is everything, and with regard to human senses and computer models that attempt to demonstrate how human sensory systems work, everything is highly complex. This includes our system of visual perception, which allows humans to interact with the dynamic world in real time. Because of biological constraints and neural processing delays, our brains must “fill in the blanks” to generate a complete picture.
Allen School professor Rajesh Rao has dedicated much of his career to the discovery of computational principles that underlie the human brain’s abilities to learn, process and store information. Recently, Rao and Allen School third-year Ph.D. student Linxing Preston Jiang have focused on understanding how our brains fill in those blanks by developing a computational model to simulate how humans process visual information. Their paper presenting their findings, “Dynamic Predictive Coding Explains Both Prediction and Postdiction in Visual Motion Perception,” will receive a Best Paper Award in the Perception and Action category at the Cognitive Science Society conference (COGSCI 2023) later this month.
“At a high level, our work supports the idea that what we consciously perceive is an edited timeline of events and not a single instant in time. This is because the brain represents events at multiple timescales,” explained Rao, the Cherng Jia and Elizabeth Yun Hwang Professor in the Allen School and UW Department of Electrical & Computer Engineering and co-director of the Center for Neurotechnology. “At the highest levels of the brain’s networks, the neural representations cover sensations in the past, present and predicted future. This explains some seemingly strange perceptual phenomena like postdiction and the flash lag illusion, where what happens in the future can affect how you perceive what is happening now.”
In their paper, Rao and Jiang hypothesized that human sensory systems encode entire sequences, or timelines, rather than just single points in time to aid perception. To test this notion, they trained a neural network model, dynamic predictive coding (DPC), to predict moving image sequences. Since DPC learns hierarchical representations of sequences, the duo found the system is able to predict the expected perceptual trajectory while compensating for transmission delays. When events deviate from the model’s expectation, the system retroactively updates its sequence representation to catch up with new observations.
In the two-level DPC model, lower-level neural states predict the next sensory input as well as the next state, while higher-level neurons predict the transition dynamics between those lower-level neural states. This enables higher-level neurons to predict entire sequences of lower-level states. This approach is grounded in predictive coding, a theory in neuroscience that suggests the brain has separate populations of neurons to encode the best prediction of what is being perceived and to identify errors in those predictions.
“Our model fits within the class of predictive coding models, which are receiving increasing attention in neuroscience as a framework for understanding how the brain works,” Rao noted. “Generative AI models like ChatGPT and GPT-4 that are trained to predict the next word in a sequence are another example of predictive coding.”
The team observed that when DPC’s visual perception relies on temporally abstracted representations — what the model has learned through minimizing prediction errors — many known predictive and postdictive phenomena in visual processing emerged from the model’s simulations. These findings support the idea that visual perception relies on the encoding of timelines rather than single points in time, which could influence the future direction of neuroscience research.
“While previous models of predictive coding mainly focused on how the visual system predicts spatially, our DPC model focuses on how temporal predictions across multiple time scales could be implemented in the visual system,” explained Jiang, who works with Rao in the Allen School’s Neural Systems Lab. “If more thoroughly validated experimentally, DPC could be used to develop solutions such as brain-computer interfaces, or BCIs, that serve as visual prostheses for the blind.”
This is just the beginning for research on DPC. A future avenue of investigation might include assessing more than the two levels of temporal hierarchies Jiang and Rao examined in the current paper with videos that have more complicated dynamics. This could facilitate comparisons of responses in DPC models with neural recordings to further explore the neural basis of multi-scale future predictions.
“Predicting the future often involves taking an animals’ own actions into account, which is a significant aspect of learning that DPC does not address,” explained Jiang. “Augmenting the DPC model with actions and using it to direct reward-based learning would be an exciting direction that has connections to reinforcement learning and motor control.”
COGSCI brings together scholars from around the world to understand the nature of the human mind. This year’s COGSCI conference will take place in Sydney, Australia.