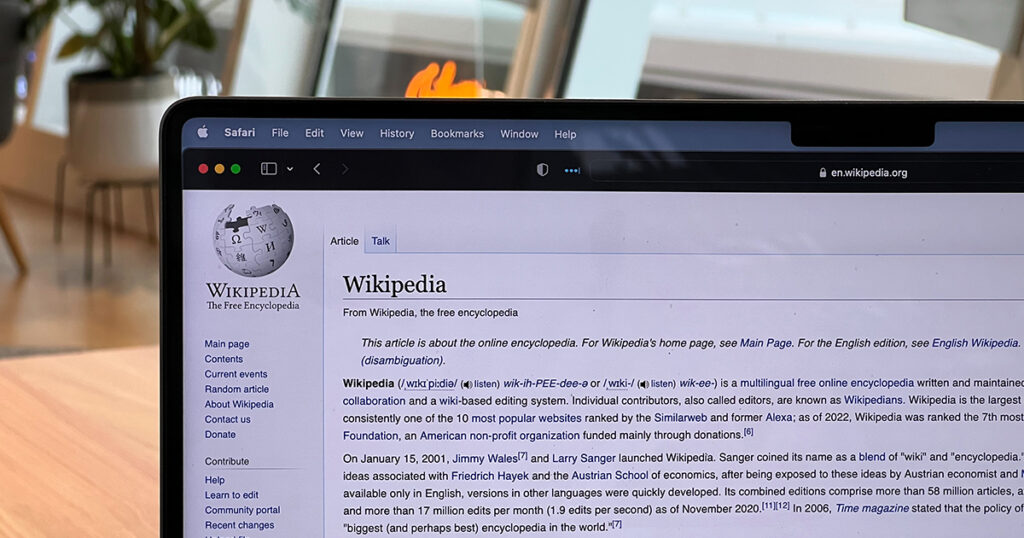
With nearly a billion unique monthly users, Wikipedia has become one of the most trusted sources of information worldwide. But while it’s considered more reliable than other internet sources, it’s not immune to bias.
Last year, a team led by Allen School professor Yulia Tsvetkov developed a new methodology for studying bias in English Wikipedia biographies, and this spring won the 2023 Wikimedia Foundation Research Award of the Year for its efforts. The team first presented its findings at The Web Conference 2022.
“Working with Wikipedia data is really exciting because there is such a robust community of people dedicated to improving the platform, including contributors and researchers,” Tsvetkov said. “In contrast, when you work with, for example, social media data, no one is going to go back and rewrite old Facebook posts. But Wikipedia editors revise articles all the time, and prior work has encouraged edit-a-thons and other initiatives for correcting biases on the platform.”
For the continuously evolving site, the research fills crucial content gaps in its data and how it is ultimately used. In the past, related studies focused mainly on one variable, binary gender, and lacked tools to isolate variables of interest, limiting the conclusions that could be drawn. For example, previous research involved comparing the complete sets of biographies for women and men in order to determine how gender influences their portrayals in these bios.
Tsvetkov’s team developed a matching algorithm to build more comprehensive and comparable sets, targeting not just gender but also other variables including race and non-binary gender. For instance, given a set of articles about women, the algorithm builds a comparison set about men that matches the initial set on as many attributes as possible (occupation, age, nationality, etc.), except the target one (gender).
The researchers could then compare statistics and language in those two sets of articles to conduct more controlled analyses of bias along a target dimension, such as gender or race. They also used statistical visualization methods to assess the quality of the matchings, supporting quantitative results with qualitative checks.
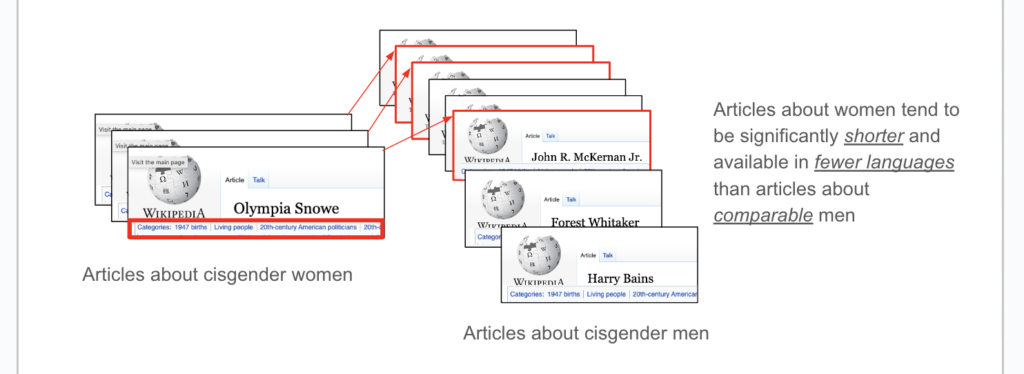
As a result, the researchers saw a significant difference when analyzing articles with and without their matching approach. When the approach was implemented, they found data confounds decreased — a boon for better evaluating bias in the future.
“We did a lot of data curation to be able to include analyses of racial bias, non-binary genders, and intersected race and gender dimensions,” said lead author Anjalie Field, a professor at Johns Hopkins University who earned her Ph.D. from Carnegie Mellon University working with Tsvetkov. “While our data and analysis focus on gender and race, our method is generalizable to other dimensions.”
Future studies could further build upon the team’s methodology, targeting biases other than gender or race. The researchers also pointed to shifting the focus from the data sets to the natural language processing (NLP) models that are deployed on them.
“As most of our team are NLP researchers, we’re also very interested in how Wikipedia is a common data source for training NLP models,” Tsvetkov said. “We can assume that any biases on Wikipedia are liable to be absorbed or even amplified by models trained on the platform.”
The study’s co-authors also included Chan Young Park, a visiting Ph.D. student from Carnegie Mellon University, and Kevin Z. Lin, an incoming professor in the University of Washington’s Department of Biostatistics. Lin earned his doctorate from Carnegie Mellon University and was a postdoc at the University of Pennsylvania when the study was published.
Learn more about the Wikimedia Research Award of the Year here, and Tsvetkov’s research group here.