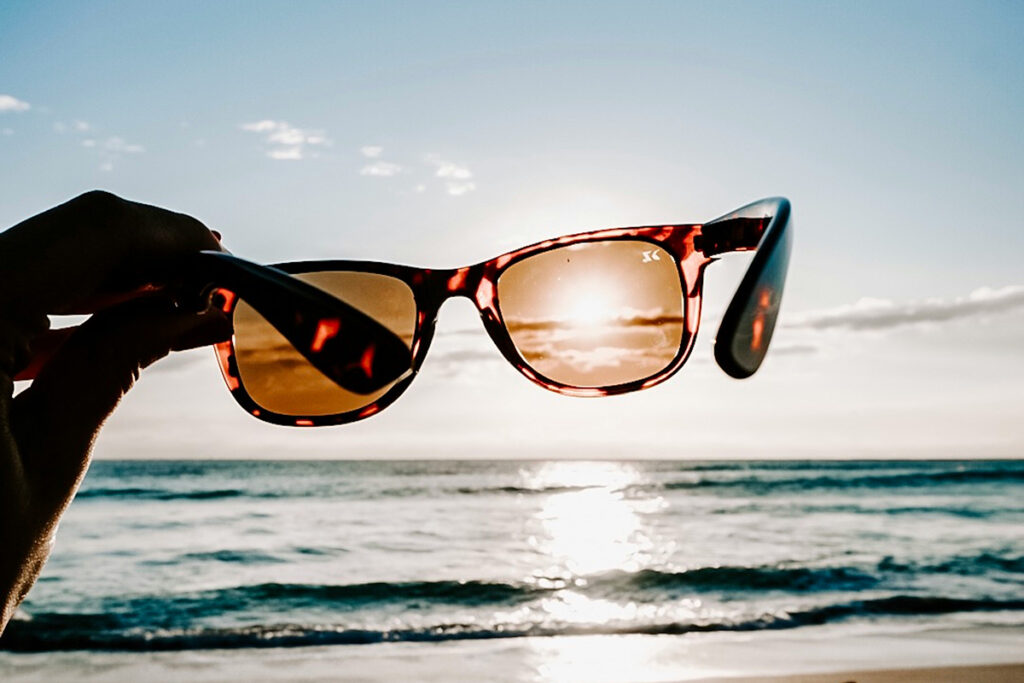
Melanoma is one of the most commonly diagnosed cancers in the United States. On the bright side, the five-year survival rate for people with this type of skin cancer is nearly 100% with early detection and treatment. And the prognosis could be even brighter with the emergence of medical-image classifiers powered by artificial intelligence, which are already finding their way into dermatology offices and consumer self-screening apps.
Such tools are used to assess whether an image depicts melanoma or some other, benign skin condition. But researchers and dermatologists have been largely in the dark about the factors which determine the models’ predictions. In a recent paper published in the journal Nature Biomedical Engineering, a team of researchers at the University of Washington and Stanford University co-led by Allen School professor Su-In Lee shed new light on the subject. They developed a framework for auditing medical-image classifiers to understand how these models arrive at their predictions based on factors that dermatologists determine are clinically significant — and where they miss the mark.
“AI classifiers are becoming increasingly popular in research and clinical settings, but the opaque nature of these models means we don’t have a good understanding of which image features are influencing their predictions,” explained lead author and Allen School Ph.D. student Alex DeGrave, who works with Lee in the AI for bioMedical Sciences (AIMS) Lab and is pursuing his M.D./Ph.D. as part of the UW Medical Scientist Training Program.
“We combined recent advances in generative AI and human medical expertise to get a clearer picture of the reasoning process behind these models,” he continued, “which will help to prevent AI failures that could influence medical decision-making.”
DeGrave and his colleagues employed an enhanced version of a technique known as Explanation by Progressive Exaggeration. Using generative AI — the same technology behind popular image generators such as DALL-E and Midjourney — they produced thousands of pairs of counterfactual images, which are images that have been altered to induce an AI model to make a different prediction from that associated with the original image. In this case, the counterfactual pairs corresponded with reference images depicting skin lesions associated with melanoma or non-cancerous conditions that may appear similar to melanoma, such as benign moles or wart-like skin growths called seborrheic keratoses.
The team trained the generator alongside a medical-image classifier to produce counterfactuals that resembled the original image, but with realistic-looking departures in pigmentation, texture and other factors that would prompt the classifier to adjudge one of the pair benign and the other malignant. They then repeated this process for a total of five AI medical-image classifiers, including an early version of an academic classifier called ModelDerm — which was subsequently approved for use in Europe — and two consumer-facing smartphone apps, Scanoma and Smart Skin Cancer Detection.
In order to infer which features contribute to a classifier’s reasoning and how, the researchers turned to human dermatologists. The physicians were asked to review the image pairs and indicate which of the counterfactuals most suggested melanoma, and then to note the attributes that differed between the images in each pair. The team aggregated those insights and developed a conceptual model of each classifier’s reasoning process based on the tendency for an attribute to sway the model towards a prediction of benign or malignant as well as the frequency with which each attribute appeared among the counterfactuals as determined by the human reviewers.
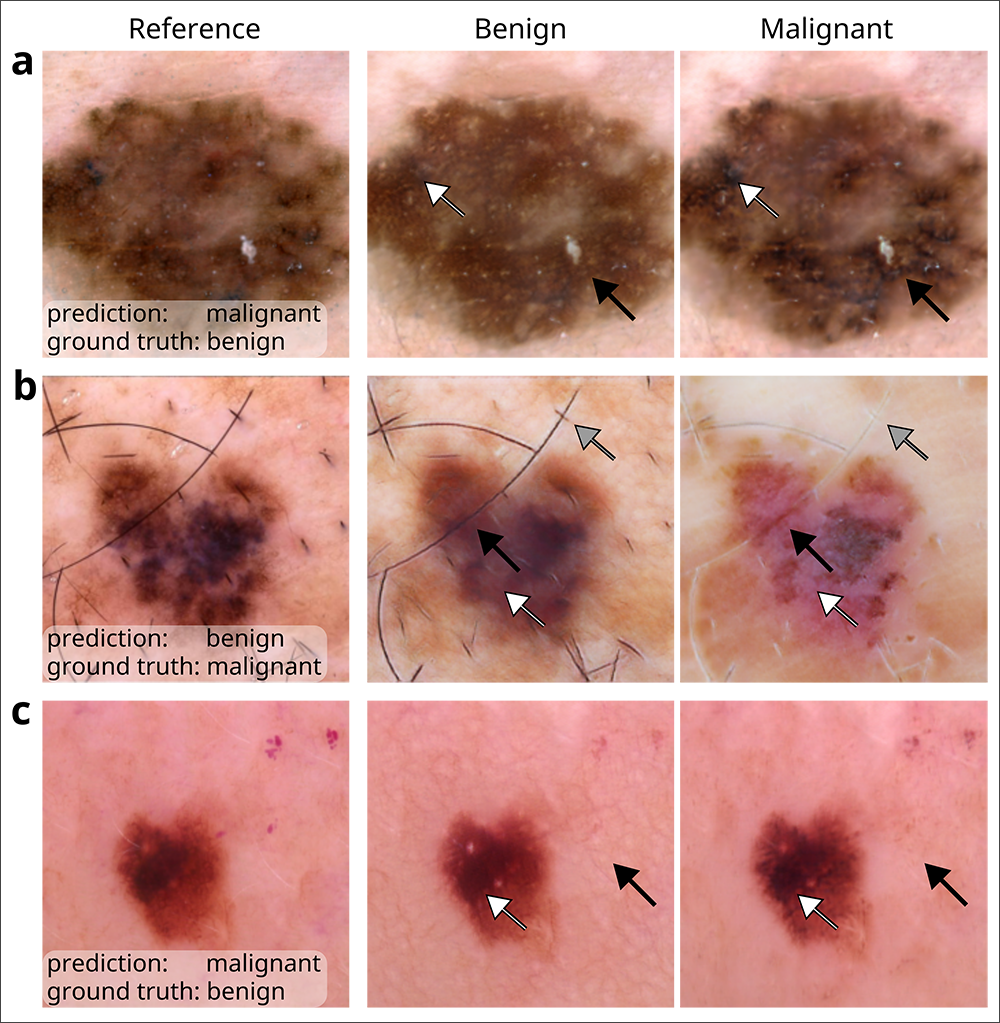
During its audit, the team determined that all five classifiers based their predictions, at least in part, on attributes that dermatologists and the medical literature have deemed medically significant. Such attributes include darker pigmentation, the presence of atypical pigmentation patterns, and a greater number of colors — each of which point to the likelihood a lesion is malignant.
In cases where the classifier failed to correctly predict the presence of melanoma, the results were mixed. In certain instances, such as when the level of pigmentation yielded an erroneous prediction of malignancy when the lesion was actually benign, the failures were deemed to be reasonable; a dermatologist would most likely have erred on the side of caution and biopsied the lesion to confirm. But in other cases, the audit revealed the classifiers were relying not so much on signal but on noise. For example, the pinkness of the skin surrounding the lesion or the presence of hair influenced the decision-making of one or more classifiers; typically, neither attribute would be regarded by dermatologists as medically relevant.
“Pinkness of the skin could be due to an image’s lighting or color balance,” explained DeGrave. “For one of the classifiers we audited, we found darker images and cooler color temperatures influenced the output. These are spurious associations that we would not want influencing a model’s decision-making in a clinical context.”
According to Lee, the team’s use of counterfactual images, combined with human annotators, revealed insights that other explainable AI techniques are likely to overlook.
“Saliency maps tend to be people’s go-to for applying explainable AI to image models because they are quite effective at identifying which regions of an image contributed most to a model’s prediction,” she noted. “For many use cases, this is sufficient. But dermatology is different, because we’re dealing with attributes that may overlap and that manifest through different textures and tones. Saliency maps are not suited to capturing these medically relevant factors.
“Our counterfactual framework can be applied in other specialized domains, such as radiology and ophthalmology, to make AI models’ predictions medically understandable,” Lee continued. “This understanding is essential to ensuring their accuracy and utility in real-world settings, where the stakes for both patients and physicians are high.”
Lee and DeGrave’s co-authors on the paper include Allen School alum and MSTP student Joseph Janizek (Ph.D., ‘22), Stanford University postdoc Zhuo Ran Cai, M.D., and Roxana Daneshjou, M.D., Ph.D., a faculty member in the Department of Biomedical Data Sciences and in Dermatology at Stanford University.
Read the full paper in Nature Biomedical Engineering and a related story by Stanford University.