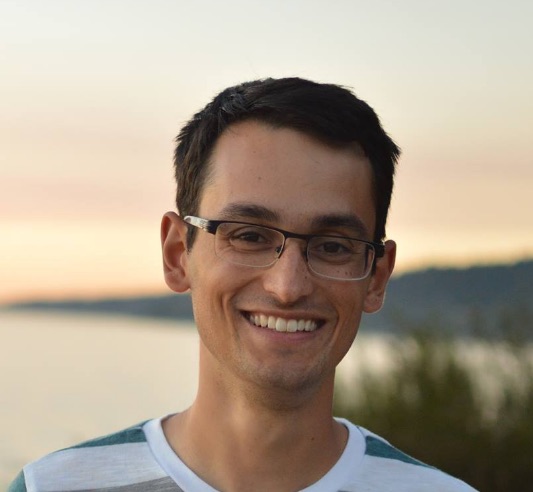
Gabriel Erion, an M.D.-Ph.D. student currently doing his Ph.D. research with Allen School professor Su-In Lee in the Artificial Intelligence for Medicine and Science (AIMS) Lab, has earned the prestigious Ruth L. Kirschstein National Research Service Award from the National Institutes of Health. The Kirschstein-NRSA, also known as the F30, was created to enhance research and clinical training of promising predoctoral students who are enrolled in a combined M.D.-Ph.D. training program and plan to pursue careers as physician-scientists.
The University of Washington is one of about 50 schools around the country that have a Medical Scientist Training Program — an M.D.-Ph.D. program supported by federal funding from the NIH. The program can last eight or more years; students spend the first two years of their training in medical school, mostly in a classroom setting. They then transition to a Ph.D. and finish the entire degree before returning to medical school for two years of primarily hospital-based clerkships. After graduating, students often split their time between research and practicing medicine. Erion was the first MSTP student at the University of Washington to join the Allen School for a Ph.D. in Computer Science & Engineering.
Erion initially planned to study biology in college and then go to medical school, but his love for building computers inspired him to take math and computer science classes, too. He switched his major to applied math, but still wanted to combine that with experience in health care.
“An M.D.-Ph.D. was one of the best ways I found to combine these fields, and the University of Washington had one of the best programs for someone with my interests,” Erion said. “Unlike many other schools, the UW program didn’t limit MSTP students to biology departments, and was supportive of my idea to do a math and computer science based Ph.D.”
Erion and Lee’s research on CoAI: Cost-Aware Artificial Intelligence for Health Care, in collaboration with Dr. Nathan White from the University of Washington Department of Emergency Medicine, helped him secure the fellowship. CoAI is a machine learning method for making cost-sensitive risk scores in clinical settings that maintains or improves accuracy while dramatically reducing the time it takes to predict a variety of patient outcomes.
Medical risk scores are used by doctors to help assess a patient’s risk of having a disease. There are hundreds of such risk scores, and they’re used for a wide range of purposes. Erion’s research focuses on three areas: predicting trauma patients’ risk of bleeding disorders, which is used by doctors to anticipate needs for resources like blood transfusions; predicting in-hospital death of patients in intensive care units, which is used to determine who needs the most attention from doctors and nurses; and predicting whether primary care patients will live for 10 years after their visit — an outcome which is not often assessed in clinics, but could be useful as a general indicator of patient health for primary care doctors.
CoAI was created to help doctors with these time-consuming risk assessments by providing a general-purpose machine learning framework for building low-cost clinical risk scores. The F30 grant will enable Erion and his team to create methodological developments and experiments to demonstrate CoAI’s value in a range of important clinical scenarios. For example, predicting a trauma patient’s risk of bleeding disorders is tedious right now with the Prediction of Acute Coagulopathy of Trauma (PACT) score, but CoAI could make it more efficient.
“The PACT score variables are some of the most time- and effort-intensive to gather, which we determined by surveying EMTs, paramedics, and nurses in our region. PACT requires blood pressure and heart rate measurements as input, as well as the Glasgow Coma Score, which is a 15-point scale that takes time and cognitive effort to calculate,” Erion explained. “We wanted to see if we could predict bleeding disorders as well as or better than PACT, while reducing the effort required for emergency medical service providers to gather necessary data for the score.”
This ease of use means the tool would be more likely to be deployed in practice, saving EMTs valuable time in the ambulance. The team conducted a survey that showed providers would prefer a risk calculator for which inputs would only take less than a minute to gather.
“We showed that CoAI can actually provide more accurate predictions than the PACT score with only 50 seconds of data-gathering, which is almost 10 times less than the PACT score was calculated to take,” Erion said. “It does this by automatically determining which variables are easy to measure, for example, data from the dispatcher that is pre-provided, or data like age that take only seconds to gather. We also demonstrated similar performance improvements for the ICU and primary care datasets.”
Prior to Erion earning the NIH fellowship, he and the CoAI team captured the Madrona Prize at the Allen School’s 2019 Research Showcase.
Congratulations, Gabe!