Artificial intelligence promises to be a powerful tool for improving the speed and accuracy of medical decision-making to improve patient outcomes. From diagnosing disease, to personalizing treatment, to predicting complications from surgery, AI could become as integral to patient care in the future as imaging and laboratory tests are today.
But as Allen School researchers discovered, AI models — like humans — have a tendency to look for shortcuts. In the case of AI-assisted disease detection, such shortcuts could lead to diagnostic errors if deployed in clinical settings.
In a new paper published in the journal Nature Machine Intelligence, a team of researchers in the AIMS Lab led by Allen School professor Su-In Lee examined multiple models recently put forward as potential tools for accurately detecting COVID-19 from chest radiography (x-ray). They found that, rather than learning genuine medical pathology, these models rely instead on shortcut learning to draw spurious associations between medically irrelevant factors and disease status. In this case, the models ignored clinically significant indicators in favor of characteristics such as text markers or patient positioning that were specific to each dataset in predicting whether an individual had COVID-19.
According to graduate student and co-lead author Alex DeGrave, shortcut learning is less robust than genuine medical pathology and usually means the model will not generalize well outside of the original setting.
“A model that relies on shortcuts will often only work in the hospital in which it was developed, so when you take the system to a new hospital, it fails — and that failure can point doctors toward the wrong diagnosis and improper treatment,” explained DeGrave, who is pursuing his Ph.D. in Computer Science & Engineering along with his M.D. as part of the University of Washington’s Medical Scientist Training Program (MSTP).
Combine that lack of robustness with the typical opacity of AI decision-making, and such a tool could go from potential life-saver to liability.
“A physician would generally expect a finding of COVID-19 from an x-ray to be based on specific patterns in the image that reflect disease processes,” he noted. “But rather than relying on those patterns, a system using shortcut learning might, for example, judge that someone is elderly and thus infer that they are more likely to have the disease because it is more common in older patients. The shortcut is not wrong per se, but the association is unexpected and not transparent. And that could lead to an inappropriate diagnosis.”
The lack of transparency is one of the factors that led DeGrave and his colleagues in the AIMS Lab to focus on explainable AI techniques for medicine and science. Most AI is regarded as a “black box” — the model is trained on massive data sets and spits out predictions without anyone really knowing precisely how the model came up with a given result. With explainable AI, researchers and practitioners are able to understand, in detail, how various inputs and their weights contributed to a model’s output.
The team decided to use these same techniques to evaluate the trustworthiness of models that had recently been touted for what appeared to be their ability to accurately identify cases of COVID-19 from chest radiography. Despite a number of published papers heralding the results, the researchers suspected that something else may be happening inside the black box that led to the models’ predictions. Specifically, they reasoned that such models would be prone to a condition known as worst-case confounding, owing to the paucity of training data available for such a new disease. Such a scenario increased the likelihood that the models would rely on shortcuts rather than learning the underlying pathology of the disease from the training data.
“Worst-case confounding is what allows an AI system to just learn to recognize datasets instead of learning any true disease pathology,” explained co-lead author Joseph Janizek, who, like DeGrave, is pursuing a Ph.D. in the Allen School in addition to earning his M.D. “It’s what happens when all of the COVID-19 positive cases come from a single dataset while all of the negative cases are in another.
“And while researchers have come up with techniques to mitigate associations like this in cases where those associations are less severe,” Janizek continued, “these techniques don’t work in situations you have a perfect association between an outcome such as COVID-19 status and a factor like the data source.”
The team trained multiple deep convolutional neural networks on radiography images from a dataset that replicated the approach used in the published papers. They tested each model’s performance on an internal set of images from that initial dataset that had been withheld from the training data and on a second, external dataset meant to represent new hospital systems. The found that, while the models maintained their high performance when tested on images from the internal dataset, their accuracy was reduced by half on the second, external set — what the researchers referred to as a generalization gap and cited as strong evidence that confounding factors were responsible for the models’ predictive success on the initial dataset. The team then applied explainable AI techniques, including generative adversarial networks (GANs) and saliency maps, to identify which image features were most important in determining the models’ predictions.
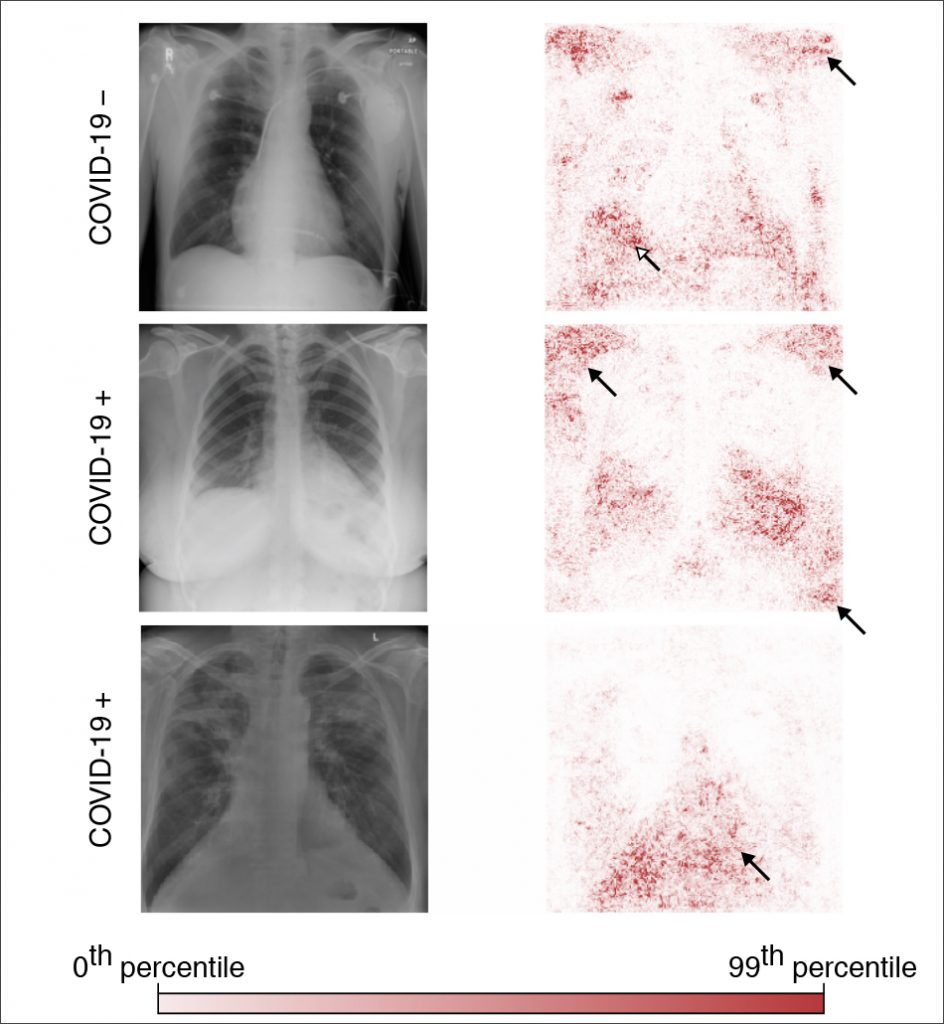
When the researchers trained the models on the second dataset, which contained images drawn from a single region and was therefore presumed to be less prone to confounding, this turned out to not be the case; even those models exhibited a corresponding drop in performance when tested on external data. These results upend the conventional wisdom that confounding poses less of an issue when datasets are derived from similar sources — and reveal the extent to which so-called high-performance medical AI systems could exploit undesirable shortcuts rather than the desired signals.
Despite the concerns raised by the team’s findings, DeGrave said it is unlikely that the models they studied have been deployed widely in the clinical setting. While there is evidence that at least one of the faulty models – COVID-Net – was deployed in multiple hospitals, it is unclear whether it was used for clinical purposes or solely for research.
“Complete information about where and how these models have been deployed is unavailable, but it’s safe to assume that clinical use of these models is rare or nonexistent,” he noted. “Most of the time, healthcare providers diagnose COVID-19 using a laboratory test (PCR) rather than relying on chest radiographs. And hospitals are averse to liability, making it even less likely that they would rely on a relatively untested AI system.”
Janizek believes researchers looking to apply AI to disease detection will need to revamp their approach before such models can be used to make actual treatment decisions for patients.
“Our findings point to the importance of applying explainable AI techniques to rigorously audit medical AI systems,” Janizek said. “If you look at a handful of x-rays, the AI system might appear to behave well. Problems only become clear once you look at many images. Until we have methods to more efficiently audit these systems using a greater sample size, a more systematic application of explainable AI could help researchers avoid some of the pitfalls we identified with the COVID-19 models,” he concluded.
Janizek, DeGrave and their AIMS Lab colleagues have already demonstrated the value of explainable AI for a range of medical applications beyond imaging. These include tools for assessing patient risk factors for complications during surgery, which appeared on the cover of Nature Biomedical Engineering, and targeting cancer therapies based on an individual’s molecular profile, as described in a paper published in Nature Communications.
“My team and I are still optimistic about the clinical viability of AI for medical imaging. I believe we will eventually have reliable ways to prevent AI from learning shortcuts, but it’s going to take some more work to get there,” Lee said. “Going forward, explainable AI is going to be an essential tool for ensuring these models can be used safely and effectively to augment medical decision-making and achieve better outcomes for patients.”
The team’s paper, “AI for radiographic COVID-19 detection selects shortcuts over signal,” is one of two from the AIMS Lab to appear in the current issue of Nature Machine Intelligence. Lee is also the senior and corresponding author on the second paper, “Improving performance of deep learning models with axiomatic attribution priors and expected gradients,” for which she teamed up with Janizek, his fellow M.D.–Ph.D. student Gabriel Erion, Ph.D. student Pascal Sturmfels, and affiliate professor Scott Lundberg (Ph.D., ‘19) of Microsoft Research to develop a robust and flexible set of tools for encoding domain-specific knowledge into explainable AI models through the use of attribution priors. Their framework supports the widespread adoption of techniques that will improve model performance and increase computational efficiency in AI for medicine and other areas of applied machine learning.
Also see a related GeekWire article here.
* Image sourced from the National Institutes of Health (NIH) Clinical Center and used with permission: Wang, X. et al. “ChestX-ray8: Hospital-scale chest X-ray database and benchmarks on weakly-supervised classification and localization of common thorax diseases.” In Proceedings of the IEEE Conference on Computer Vision and Pattern Recognition (CVPR). 2017. https://nihcc.app.box.com/v/ChestXray-NIHCC
^ Figure 2a (bottom) adapted with permission from Winther, H. et al. COVID-19 image repository. figshare. https://doi.org/10.6084/m9.figshare.12275009