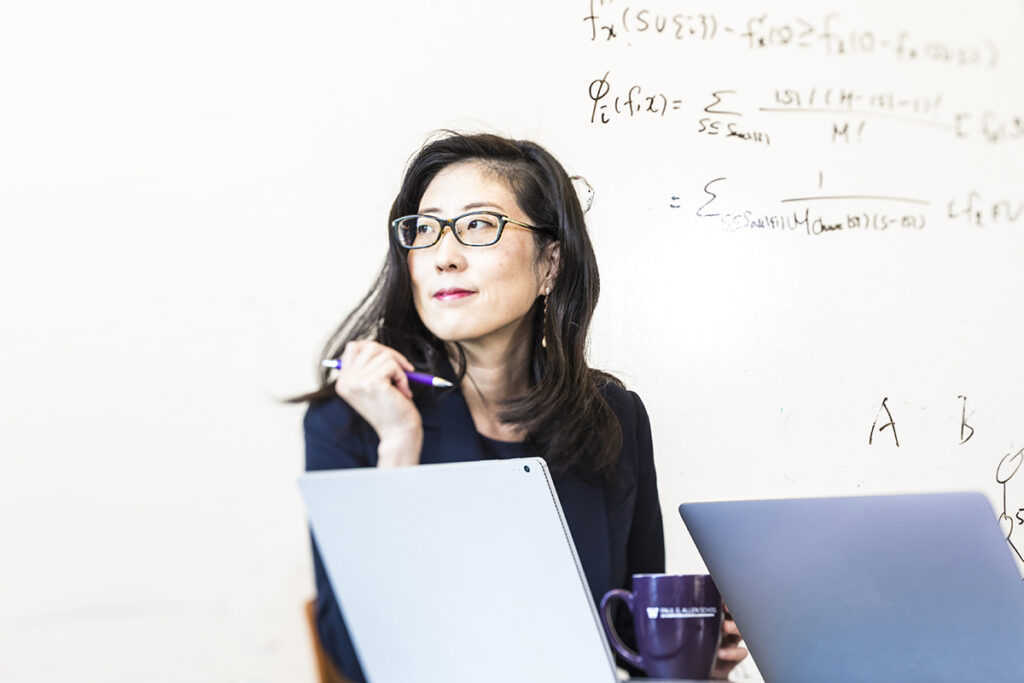
For as long as she can remember, Allen School professor Su-In Lee wanted to be a scientist and professor when she grew up. Her father, who majored in marine shipbuilding engineering, would sit her down at their home in Korea with a pencil and paper to teach her math. Those childhood lessons instilled in Lee not just a love of the subject matter but also of teaching; her first pupil was her younger brother, with whom she shared what she had learned about arithmetic and geometry under her father’s tutelage.
Fast forward a few decades, and Lee is putting those lessons to good use in training the next generation of scientists and engineers while advancing explainable artificial intelligence for biomedical applications. She is also adding up the accolades in response to her work. In February, the International Society for Computational Biology recognized Lee with its 2024 ISCB Innovator Award, given to a mid-career scientist who has consistently made outstanding contributions to the field of computational biology and continues to forge new directions; last month, the American Institute for Medical and Biological Engineering inducted her into the AIMBE College of Fellows — putting her among the top 2% of medical and biological engineers. Yesterday, the Ho-Am Foundation announced Lee as the 2024 Samsung Ho-Am Prize Laureate in Engineering for her pioneering contributions to the field of explainable AI.
As the saying goes, good things come in threes.
“This is an incredible honor for me, and I’m deeply grateful for the recognition,” said Lee, who holds the Paul G. Allen Professorship in Computer Science & Engineering and directs the AI for bioMedical Sciences (AIMS) Lab at the University of Washington. “There are so many deserving researchers, I am humbled to have been chosen. One of the most rewarding aspects of my role as a faculty member and scientist is serving as an inspiration for young people. As AI continues to transform science and society, I hope this inspires others to tackle important challenges to improve health for everyone.”
The Ho-Am Prize, which is often referred to as the “Korean Nobel Prize,” honors people of Korean heritage who have made significant contributions in academics, the arts and community service or to the welfare of humanity through their professional achievements. Previous laureates include Fields Medal-winning mathematician June Huh and Academy Award winning director Joon-ho Bong of “Parasite” fame. In addition to breaking new ground through her work, Lee has broken the glass ceiling: She is the first woman to receive the engineering prize in the award’s 34-year history and, still in her 40s, one of the youngest recipients in that category — a testament to the outsized impact she has made so early in her career.
From 1-2-3 to A-B-C
As a child, she may have learned to love her 1-2-3s; as a researcher, Lee became more concerned with her A-B-Cs: AI, biology and clinical medicine.
“The future of medicine hinges on the convergence of these disciplines,” she said. “As electronic health records become more prevalent, so too will omic data, where AI will play a pivotal role.”
Before her arrival at Stanford to pursue her Ph.D., the “C” could have stood for “cognition,” which marked her first foray into researching AI models like deep neural networks. For her undergraduate thesis, she developed a DNN for hand-written digit recognition that won the 2000 Samsung Humantech Paper Award. In the Stanford AI Lab, Lee shifted away from cognition and toward computational molecular biology, enticed by the prospect of identifying cures for diseases such as Alzheimer’s. She continued to be captivated by such questions after joining the Allen School faculty in 2010.
Six years after her arrival, Lee’s research took an unexpected — but welcome — turn when Gabriel Erion Barner knocked on her office door. Erion Barner was a student in the UW’s Medical Scientist Training Program, or MSTP, and he had a proposition.
“MSTP students combine a medical degree with a Ph.D. in a complementary field, and they are amazing,” said Lee. “Gabe was excited about AI’s potential in medicine, so he decided he wanted to do a Ph.D. in Computer Science & Engineering working with me. There was just one problem: our Ph.D. program didn’t have a process to accommodate students like Gabe. So, we created one.”
Erion Barner formally enrolled the following year and became the first MSTP student to graduate with an Allen School degree, in the spring of 2021. But he wouldn’t be the last. Joseph Janizek (Ph.D., ‘22) and current M.D. student Alex DeGrave subsequently sought out Lee as an advisor. Erion Barner has since moved on to Harvard Medical School to complete his medical residency, while Janizek is about to do the same at Lee’s alma mater, Stanford.
Meanwhile, Lee’s own move into the clinical medicine aspect of the A-B-Cs was complete.
Getting into SHAP
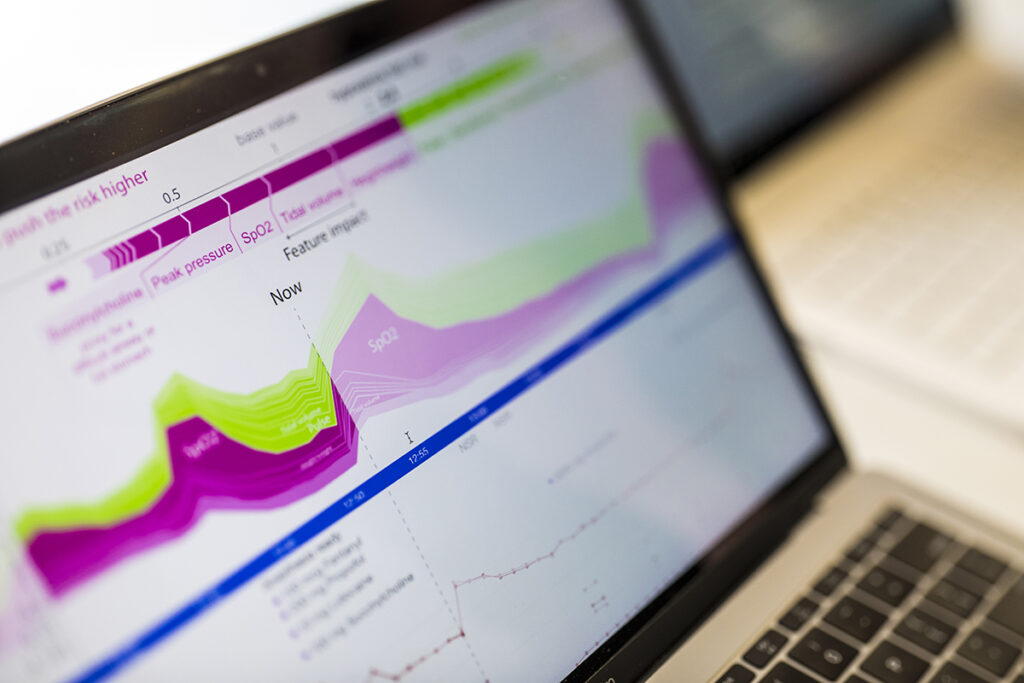
Beyond the A-B-Cs, Lee subscribes to a philosophy she likens to latent factor theory. A term borrowed from machine learning, latent factor theory posits that there are underlying — and unobserved — factors that impact upon the observable ones. Lee applies this theory when selecting the research questions in which she will invest her time. It’s part of her quest to identify that underlying factor which will transcend multiple problems, domains and disciplines.
So, when researchers began applying AI to medicine, Lee was less interested in making the models’ predictions more accurate in favor of understanding why they made the predictions they did in the first place.
“I just didn’t want to do it,” she said of pursuing the accuracy angle. “Of course we need the models to be accurate, but why was a certain prediction made? I realized that addressing the black box of AI — those latent factors — would be helpful for clinical decision-making and for clinicians’ perceptions of whether they could trust the model or not.” This principle, she noted, extends beyond medical contexts to areas like finance.
Lee discovered that the questions she raised around transparency and interpretability were the same questions circulating in the medical community. “They don’t just want to be warned,” she said. “They want to know the reasons behind the warning.”
In 2018, Lee and her team began to shine a lot on the models’ reasoning. Their first clinical paper, appearing on the cover of Nature Biomedical Engineering, described a novel framework for not only predicting but also providing real-time explanations for a patient’s risk of developing hypoxemia during surgery. The framework, called Prescience, relied on SHAP values — short for SHapley Additive exPlanations — which applied a game theoretic approach to explain the weighted outputs of a model. The paper, which is broadly applicable to many domains, has garnered more than 1,300 citations. In follow-up work, Lee and her team unveiled CoAI, or Cost-Aware Artificial Intelligence, which applied Shapley values to prioritize which patient risk factors to evaluate in emergency or critical care scenarios given a budget of time, resources or both.
AI under the microscope
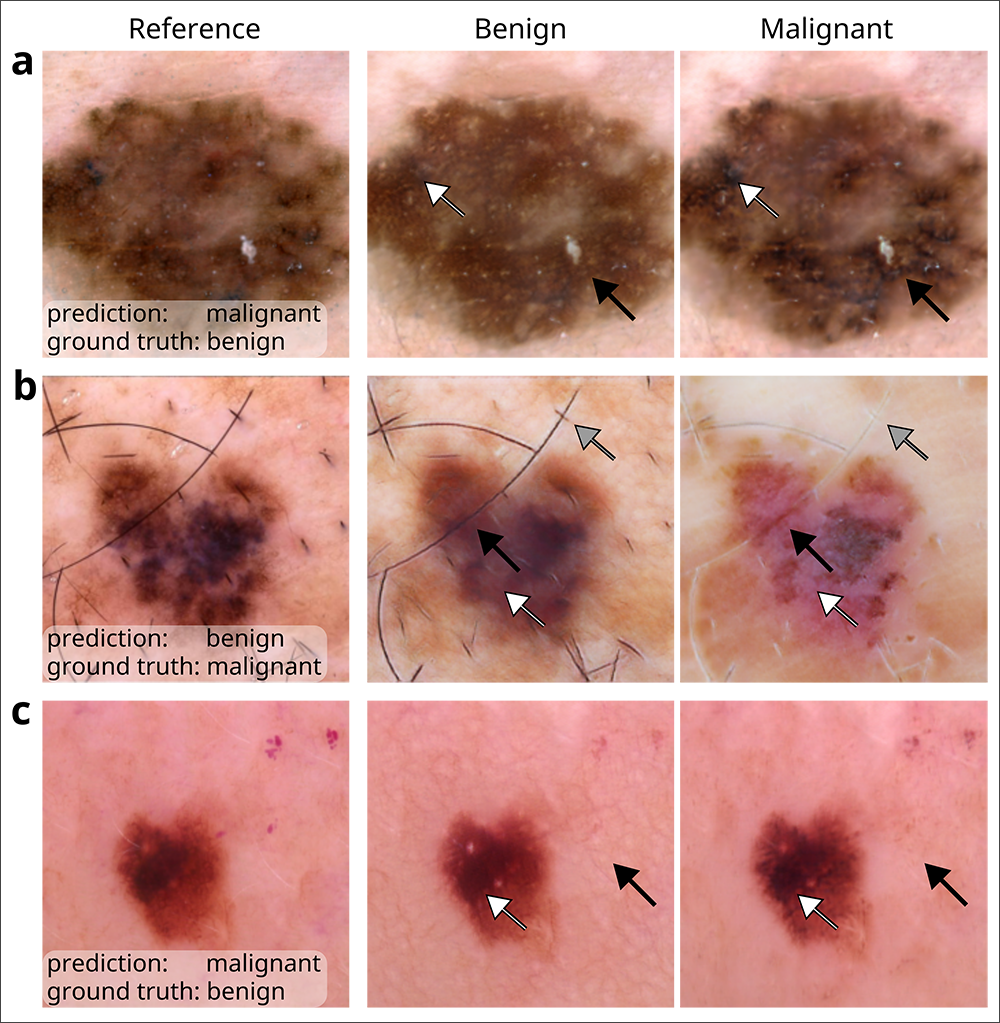
Lee and her collaborators subsequently shifted gears in the direction of developing fundamental AI principles and techniques that could transcend any single clinical or research question. Returning to her molecular biology roots, Lee was curious about how she could use explainable AI to solve common problems in analyzing single-cell datasets to understand the mechanisms and treatment of disease. But to do that, researchers would need to run experiments in which they disentangle variations in the target cells from those in the control dataset to identify which factors are relevant and which merely confound the results. To that end, Lee and her colleagues developed ContrastiveVI, a deep learning framework for applying a technique known as contrastive analysis to single-cell datasets. The team published their framework in Nature Methods.
“By addressing contrastive scientific questions, we can help solve many problems,” Lee explained. “Our methods enable us to handle these nuanced datasets effectively.”
Up to that point, the utility of CA in relation to single cell data was limited; for once, latent factors — in this case, the latent variables typically used to model all variations in the data — worked against the insights Lee sought. ContrastiveVI solves this problem by separating those latent variables, which are shared across both the target and control datasets, from the salient variables exclusive to the target cells. This enables comparisons of, for example, the differences in gene expression between diseased and healthy tissue, the body’s response to pathogens or drugs, or CRISPR-edited versus unedited genomes.
Lee and her colleagues also decided to put medical AI models themselves under the microscope, applying more scrutiny to their predictions by developing techniques to audit their performance in domains ranging from dermatology to radiology. As they discovered, even when a model’s predictions are accurate, they should be treated with a healthy dose of skepticism.
“I’m particularly drawn to this direction because it underscores the importance of understanding AI models’ reasoning processes before blindly using them — a principle that extends across disciplines, from single-cell foundation models, to drug discovery, to clinical trial identification,” she said.
As one example, Lee and her team revealed how models that analyze chest x-rays to predict whether a patient has COVID-19 tend to rely on so-called shortcut learning, which leads them to base their predictions on spurious factors rather than genuine medical pathology. The journal Nature highlighted their work the following year. More recently, Lee co-authored a paper in Nature Biomedical Engineering that leveraged generative AI to audit medical-image classifiers used for predicting melanoma, finding that they rely on a mix of clinically significant factors and spurious associations. The team’s method, which favored the use of counterfactual images over conventional saliency maps to make the image classifiers’ predictions medically understandable, could be extended to other domains such as radiology and ophthalmology. Lee recently published her futuristic perspectives on the clinical potential of counterfactual AI in The Lancet.
Going forward, Lee is “really excited” about tackling the black-box nature of medical-image classifiers by automatically annotating semantically meaningful concepts using image-text foundation models. In fact, she has a paper on this very topic that is slated to be published in Nature Medicine this spring.
“All research topics hold equal fascination for me, but if I had to choose one, our AI model auditing framework stands out,” Lee said. “This unique approach can be used to uncover flaws in the reasoning process of AI models, which could solve a lot of society’s concerns about AI. We humans have a tendency to fear the unknown, but our work has demonstrated that AI is knowable.”
Live long and prosper
From life-and-death decisionmaking in the ER, to a more nuanced approach to analyzing life and death: One of the topics Lee has investigated recently concerns applying AI techniques to massive amounts of clinical data to understand people’s biological ages. The ENABL Age framework — which stands for ExplaiNAble BioLogical Age — applied explainable AI techniques to all-cause and cause-specific mortality to predict individuals’ biological ages and identify the underlying risk factors that contributed to those predictions to potentially inform clinical decision-making. The paper was featured on the cover of Lancet Healthy Longevity last December.
Lee hopes to build on this work to uncover the drivers of aging as well as the underlying mechanisms of rejuvenation — a topic she looks forward to exploring with her peers at a workshop she is co-leading in May. She is also keen to continue applying AI insights to identify therapeutic targets for Alzheimer’s disease, which is one of the 10 deadliest diseases in the U.S., as well as other neurodegenerative conditions. Her prior work on this topic was published in Nature Communications in 2021 and Genome Biology in 2023.
Even with AI’s flaws — some of which she herself has uncovered — Lee believes that it will prove to be a net benefit to society.
“Like other technologies, AI carries risks,” Lee acknowledged. “But those risks can be mitigated through the use of complementary technologies such as explainable AI that allow us to interpret complex AI models to promote transparency, accountability, and ultimately, trust.”
If Lee’s father, who passed away in 2013, could see her now, he would no doubt be impressed with how those early math lessons added up.