The Allen School is preparing to welcome five faculty hires in 2019-2020 who will enhance the University of Washington’s leadership at the forefront of computing innovation, including robot learning for real-world systems, machine learning and its implications for society, online discussion systems that empower users and communities, and more. Meet Byron Boots, Kevin Lin, Jamie Morgenstern, Alex Ratner, and Amy Zhang — the outstanding scholars set to expand the school’s research and teaching in exciting new directions while building on our commitment to excellence, mentorship, and service:
Byron Boots, machine learning and robotics
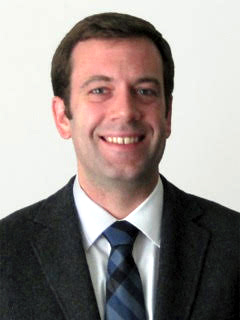
Former Allen School postdoc Byron Boots returns to the University of Washington this fall after spending five years on the faculty of Georgia Institute of Technology, where he leads the Georgia Tech Robot Learning Lab. Boots, who earned his Ph.D. in Machine Learning from Carnegie Mellon University, advances fundamental and applied research at the intersection of artificial intelligence, machine learning, and robotics. He focuses on the development of theory and systems that tightly integrate perception, learning, and control while addressing problems in computer vision, system identification, state estimation, localization and mapping, motion planning, manipulation, and more. Last year, Boots received a CAREER Award from the National Science Foundation for his efforts to combine the strengths of hand-crafted, physics-based models with machine learning algorithms to advance robot learning.
Boots’ goal is to make robot learning more efficient while producing results that are both interpretable and safe for use in real-world systems such as mobile manipulators and high-speed ground vehicles. His work draws upon and extends theory from nonparametric statistics, graphical models, neural networks, non-convex optimization, online learning, reinforcement learning, and optimal control. One of the areas in which Boots has made significant contributions is imitation learning (IL), a promising avenue for accelerating policy learning for sequential prediction and high-dimensional robotics control tasks more efficiently than conventional reinforcement learning (RL). For example, Boots and colleagues at Carnegie Mellon University introduced a novel approach to imitation learning, AggreVaTeD, that allows for the use of expressive differentiable policy representations such as deep networks while leveraging training-time oracles. Using this method, the team showed that it could achieve faster and more accurate solutions with less training data — formally demonstrating for the first time that IL is more effective than RL for sequential prediction with near-optimal oracles.
Following up on this work, Boots and graduate student Ching-An Cheng produced new theoretical insights into value aggregation, a framework for solving IL problems that combines data aggregation and online learning. As a result of their analysis, the duo was able to debunk the commonly held belief that value aggregation always produces a convergent policy sequence while identifying a critical stability condition for convergence. Their work earned a Best Paper Award at the 21st International Conference on Artificial Intelligence and Statistics (AISTATS 2018).
Driven in part by his work with highly dynamic robots that engage in complex interactions with their environment, Boots has also advanced the state of the art in robotic control. Boots and colleagues recently developed a learning-based framework, Dynamic Mirror Descent Model Predictive Control (DMD-MPC), that represents a new design paradigm for model predictive control (MPC) algorithms. Leveraging online learning to enable customized MPC algorithms for control tasks, the team demonstrated a set of new MPC algorithms created with its framework on a range of simulated tasks and a real-world aggressive driving task. Their work won Best Student Paper and was selected as a finalist for Best Systems Paper at the Robotics: Science and Systems (RSS 2019) conference this past June.
Among Boots’ other recent contributions is the Gaussian Process Motion Planner algorithm, a novel approach to motion planning viewed as probabilistic inference that was designated “Paper of the Year” for 2018 by the International Journal of Robotics Research, and a robust, precise force prediction model for learning tactile perception developed in collaboration with NVIDIA’s Seattle Robotics Research Lab, where he teamed up with his former postdoc advisor, Allen School professor Dieter Fox.
Kevin Lin, computer science education
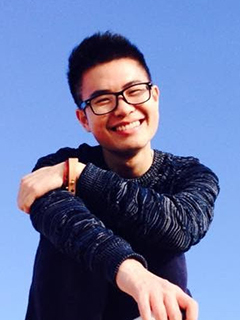
Kevin Lin arrives at the Allen School this fall as a lecturer specializing in introductory computer science education and broadening participation at the K-12 and post-secondary levels. Lin earned his master’s in Computer Science and bachelor’s in Computer Science and Cognitive Science from the University of California, Berkeley. Before joining the faculty at the Allen School, Lin spent four years as a teaching assistant (TA) at Berkeley, where he taught courses in introductory programming, data structures, and computer architecture. As a head TA for all three of these courses, he coordinated roughly 40 to 100 TAs per semester and focused on improving the learning experience both inside and outside the classroom.
Lin’s experience managing courses with over 1,400 enrolled students spurred his interest in using innovative practices and software tools to improve student engagement and outcomes and to attract and retain more students in the field. While teaching introductory programming at Berkeley, Lin helped introduce and expand small-group mentoring sections led by peer students who previously took the course. These sections offered a safe environment in which students could ask questions and make mistakes outside of the larger lecture. He also developed an optimization algorithm for assigning students to these sessions, with an eye toward improving not only their academic performance but also their sense of belonging in the major. As president of Computer Science Mentors, Lin grew the organization from 100 mentors to more than 200 and introduced new opportunities for mentor training and professional development.
In addition to supporting peer-to-peer mentoring, Lin uses software to effectively share feedback with students as a course progresses. For example, he helped build a system that automatically prompts students to interactively explore at predetermined intervals the automated checks which will be applied to their solution. By giving students an opportunity to compare their own expectations for a program’s behavior against the real output, this approach builds students’ confidence in their work while reducing their need to ask for clarifications on an assignment. He also developed a system for sharing individualized, same-day feedback with students about in-class quiz results to encourage them to revisit and learn from their mistakes. Lin also has taken a research-based approach to identifying the most effective interventions that instructors can use to improve student performance.
Outside of the classroom, Lin has led efforts to expand K-12 computer-science education through pre-service teacher training and the incorporation of CS principles in a variety of subjects. He has also been deeply engaged in efforts to improve undergraduate education as a coordinator of Berkeley’s EECS Undergraduate Teaching Task Force.
Jamie Morgenstern, machine learning and theory of computation
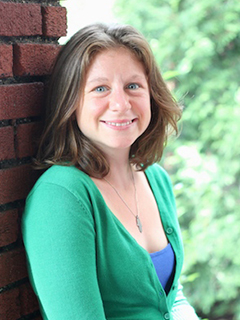
Jamie Morgenstern joins the Allen School faculty this fall from the Georgia Institute of Technology, where she is a professor in the School of Computer Science. Her research explores the social impact of machine learning and how social behavior influences decision-making systems. Her goal is to build mechanisms for ensuring fair and equitable application of machine learning in domains people interact with every day. Other areas of interest algorithmic game theory and its application to economics — specifically, how machine learning can be used to make standard economic models more realistic and relevant in the age of e-commerce. Previously, Morgenstern spent two years as a Warren Fellow of Computer Science and Economics at the University of Pennsylvania after earning her Ph.D. in Computer Science from Carnegie Mellon University.
Machine learning has emerged as an indispensable tool for modern communication, financial services, medicine, law enforcement, and more. The application of ML in these system introduces incredible opportunities for more efficient use of resources. However, introducing these powerful computational methods in dynamic, human-centric environments may exacerbate inequalities present in society, as they may well have uneven performance for different demographic groups. Morgenstern terms this phenomenon “predictive inequity.” It manifests in a variety of online settings — from determining which discounts and services are offered, to deciding which news articles and ads people see. Predictive inequity may also affect people’s safety in the physical world, as evidenced by what Morgenstern and her colleagues found in an examination of object detection systems that interpret visual scenes to help prevent collisions involving vehicles, pedestrians, or elements of the environment. The team found that object detection’s performance varies when measured on pedestrians with differing skin types, independent of time of day or several other potentially mitigating factors. In fact, all of the models Morgenstern and her colleagues analyzed exhibited poorer performance in detecting people with darker skin types — defined as Fitzpatrick skin types between 4 and 6 — than pedestrians with lighter skin types. Their results suggest, if these discrepancies in performance are not addressed, certain demographic groups may face greater risk of fatality from object recognition failures.
Spurred on by these and other findings, Morgenstern aims to develop techniques for building predictive equity into machine learning models to ensure they treat members of different demographic groups with similarly high fidelity. For example, she and her colleagues developed a technique for increasing fairness in principal component analysis (PCA), a popular method of dimensionality reduction in the sciences that is intended to reduce bias in datasets but that, in practice, can inadvertently introducing new bias. In other work, Morgenstern and colleagues developed algorithms for incorporating fairness constraints in spectral clustering and also in data summarization to ensure equitable treatment of different demographic groups in unsupervised learning applications.
Morgenstern also aims to understand how social behavior and self-interest can influence machine learning systems. To that end, she analyzes how systems can be manipulated and designs systems to be robust against such attempts at manipulation, with potential applications in lending, housing, and other domains that rely on predictive models to determine who receives a good. In one ongoing project, Morgenstern and her collaborators have explored techniques for using historical bidding data, where bidders may have attempted to game the system, to optimize revenue while incorporating incentive guarantees. Her work extends to making standard economic models more realistic and robust in the face of imperfect information. For example, Morgenstern is developing a method for designing near-optimal auctions from samples that achieve high welfare and high revenue while balancing supply and demand for each good. In a previous paper selected for a spotlight presentation at the 29th Conference on Neural Information Processing Systems (NeurIPS 2015), Morgenstern and Stanford University professor Tim Roughgarden borrowed techniques from statistical learning theory to present an approach for designing revenue-maximizing auctions that incorporates imperfect information about the distribution of bidders’ valuations while at the same time providing robust revenue guarantees.
Alex Ratner, machine learning, data management, and data science
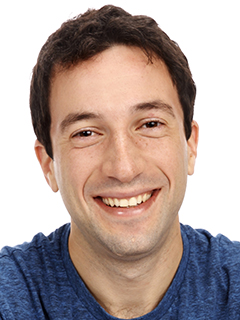
Alexander Ratner will arrive at the University of Washington in fall 2020 from Stanford University, where he will have completed his Ph.D. working with Allen School alumnus Christopher Ré (Ph.D., ‘09). Ratner’s research focuses on building new high level systems for machine learning based around “weak supervision,” enabling practitioners in a variety of domains to use less precise, higher-level inputs to generate dynamic and noisy training sets on a massive scale. His work aims to overcome a key obstacle to the rapid development and deployment of state-of-the-art machine learning applications — namely, the need to manually label and manage training data — using a combination of algorithmic, theoretical, and systems-related techniques.
Ratner led the creation and development of Snorkel, an open-source system for building and managing training datasets with weak supervision that earned a “Best of” Award at the 44th International Conference on Very Large Databases (VLDB 2018). Snorkel is the first system of its kind that focuses on enabling users to build and manipulate training datasets programmatically, by writing labeling functions, instead of the conventional and painstaking process of labeling data by hand. The system automatically reweights and combines the noisy outputs of those labeling functions before using them to train the model — a process that enables users, including subject-matter experts such as scientists and clinicians, to build new machine learning applications in a matter of days or weeks, as opposed to months or years. Technology companies, including household names such as Google, Intel, and IBM, have deployed Snorkel for content classification and other tasks, while federal agencies such as the U.S. Department of Veterans Affairs (VA) and the Food and Drug Administration (FDA) have used it for medical informatics and device surveillance tasks. Snorkel is also deployed as part of the Defense Advanced Research Projects Agency (DARPA) MEMEX program, an initiative aimed at advancing online search and content indexing capabilities to combat human trafficking; in a variety of medical informatics and triaging projects in collaboration with Stanford Medicine, including two that were presented in Nature Communications papers this year; and a range of other open source use cases.
Building on Snorkel’s success, Ratner and his collaborators subsequently released Snorkel MeTaL, now integrated into the latest Snorkel v0.9 release, to apply the principle of weak supervision to the training of multi-task learning models. Under the Snorkel MeTaL framework, practitioners write labeling functions for multiple, related tasks, and the system models and integrates various weak supervision sources to account for varied — and often, unknown — levels of granularity, correlation, and accuracy. The team approached this integration in the absence of labels as a matrix completion-style problem, introducing a simple yet scalable algorithm that leverages the dependency structure of the sources to recover unknown accuracies while exploiting the relationship structure between tasks. The team recently used Snorkel MeTaL to help achieve a state-of-the-art result on the popular SuperGLUE benchmark, demonstrating the effectiveness of programmatically building and managing training data for multi-task models as a new focal point for machine learning developers.
Another area of machine learning in which Ratner has developed new systems, algorithms, and techniques is data augmentation, a technique for artificially expanding or reshaping labeled training datasets using task-specific data transformations in which class labels are preserved. Data augmentation is an essential tool for training modern machine learning models, such as deep neural networks, that require massive labeled datasets. As a manual task, it requires time-intensive manual tuning to achieve the compositions required for high-quality results, while a purely automated approach tends to produce wide variations in end performance. Ratner co-led the development of a novel method for data augmentation that leverages user domain knowledge combined with automation to achieve more accurate results in less time. Ratner’s approach enables subject-matter experts to specify black-box functions that transform data points, called transformation functions (TFs), then applies a generative sequence model over the specified TFs to produce realistic and diverse datasets. The team demonstrated the utility of its approach by training its model to rotate, rescale, and move tumors in ultrasound images — a result that led to real-world improvements in mammography screening and other tasks.
Amy Zhang, human-computer interaction and social computing
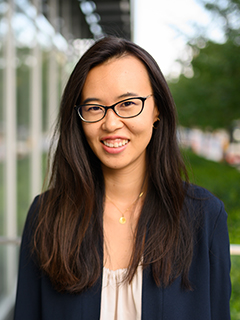
Amy Zhang will join the Allen School faculty in fall 2020 after completing her Ph.D. in Computer Science at MIT. Zhang focuses on the design and development of online discussion systems and computational techniques that empower users and communities by giving them the means to control their online experiences and the flow of information. Her work, which blends deep qualitative needfinding with large-scale quantitative analysis towards the design and development of new user-facing systems, spans human-computer interaction, computational social science, natural language processing, machine learning, data mining, visualization, and end-user programming. Zhang recently completed a fellowship with the Berkman Klein Center for Internet & Society at Harvard University. She was previously named a Gates Scholar at the University of Cambridge, where she earned her master’s in Computer Science.
Online discussion systems have a profound impact on society by influencing the way we communicate, collaborate, and participate in public discourse. Zhang’s goal is to enable people to effectively manage and extract useful knowledge from large-scale discussions, and to provide them with tools for customizing their online social environments to suit their needs. For example, she worked with Justin Cranshaw of Microsoft Research to develop Tilda, a system for collaboratively tagging and summarizing group chats on platforms like Slack. Tilda — a play on the common expression “tl;dr,” or “too long; didn’t read” — makes it easier for participants to enrich and extract meaning from their conversations in real time as well as catch up on content they may have missed. The team’s work earned a Best Paper Award at the 21st ACM Conference on Computer-Supported Cooperative Work and Social Computing (CSCW 2018).
The previous year at CSCW, Zhang and her MIT colleagues presented Wikum, a system for creating and aggregating summaries of large, threaded discussions that often contain a high degree of redundancy. The system, which draws its name from a portmanteau of “wiki” and “forum,” employs techniques from machine learning and visualization, as well as a new workflow Zhang developed called recursive summarization. This approach produces a summary tree that enables readers to explore a discussion and its subtopics at multiple levels of detail according to their interests. Wikipedia editors have used the Wikum prototype to resolve content disputes on the site. It was also selected by the Collective Intelligence for Democracy Conference in Madrid as a tool for engaging citizens on discussions of city-wide proposals in public forums such as Decide Madrid.
Zhang has also tackled the dark side of online interaction, particularly email harassment. In a paper that appeared at last year’s ACM Conference on Human Factors in Computing Systems (CHI 2018), Zhang and her colleagues introduced a new tool, Squadbox, that enables recipients to coordinate with a supportive group of friends — their “squad” — to moderate the offending messages. Squadbox is a platform for supporting highly customized, collaborative workflows that allow squad members to intercept, reject, redirect, filter, and organize messages on the recipient’s behalf. This approach, for which Zhang coined the term “friendsourced moderation,” relieves the victims of the emotional and temporal burden of responding to repeated harassment. A founding member of the Credibility Coalition, Zhang has also contributed to efforts to develop transparent, interoperable standards for determining the credibility of news articles using a combination of article text, external sources, and article metadata. As a result of her work, Zhang was featured by the Poynter Institute as one of six academics on the “frontlines of fake news research.”
This latest group of educators and innovators join an impressive — and impressively long — list of recent additions to the Allen School faculty who have advanced UW’s reputation across the field. They include the addition of Tim Althoff, whose research applies data science to advance human health and well-being; Hannaneh Hajishirzi, an expert in natural language processing; René Just, whose research combines software engineering and security; Rachel Lin and Stefano Tessaro, who introduced exciting new expertise in cryptography; Ratul Mahajan, a prolific researcher in networking and cloud computing; Sewoong Oh, who is advancing the frontiers of theoretical machine learning; Hunter Schafer and Brett Wortzman, who support student success in core computer science education; and Adriana Schulz, who is focused on computational design for manufacturing to drive the next industrial revolution. Counting the latest arrivals, the Allen School has welcomed a total of 22 new faculty members in the past three years.
A warm Allen School welcome to Alex, Amy, Byron, Jamie, and Kevin!